- Home
- Training Data Types
Training Data Types
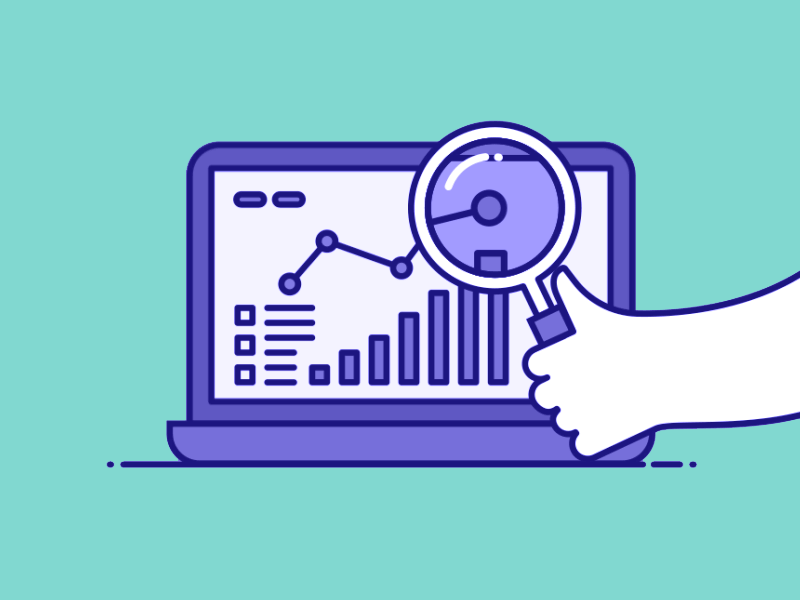
Diverse training data types in business sales, such as text, audio, and image data, refine machine learning models, optimize personalization, and enhance decision-making, ultimately driving revenue growth.
Service Types
Training Data Types
Why Choose Us
With great features comes great success.
We give top-notch services to our clients and a dedicated FTP
We handle difficult projects with ease and are quite conscientious about meeting our deadlines.
Large international organizations are among our oldest and most renowned clients
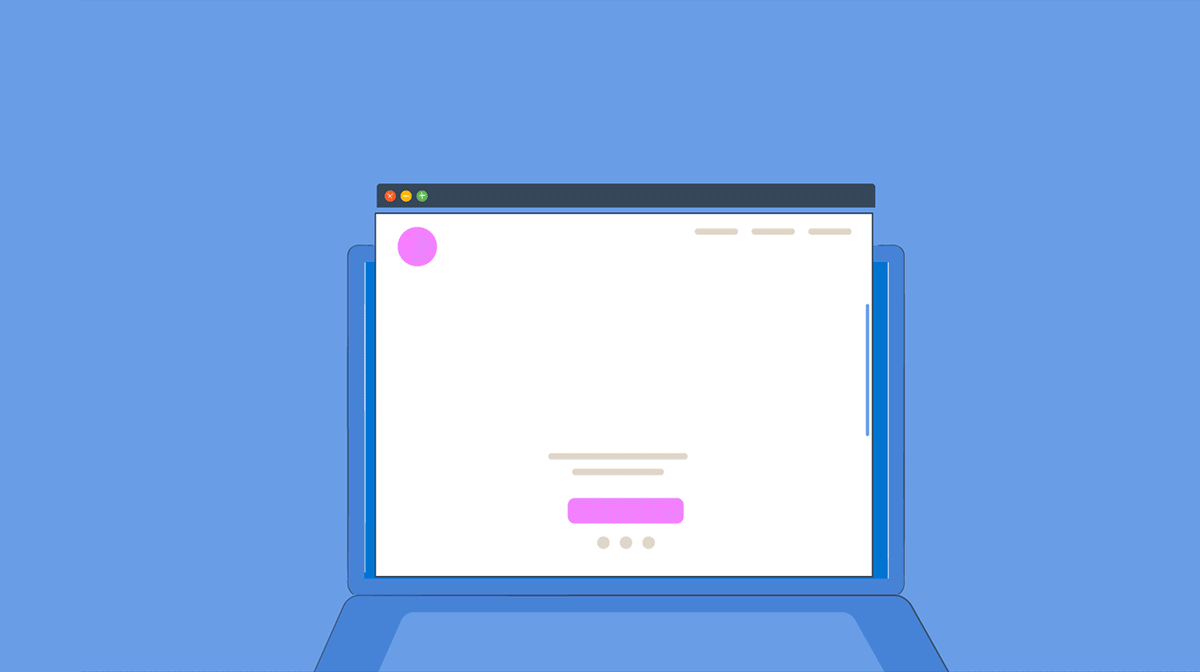
CSAT: 98.7
What they say?
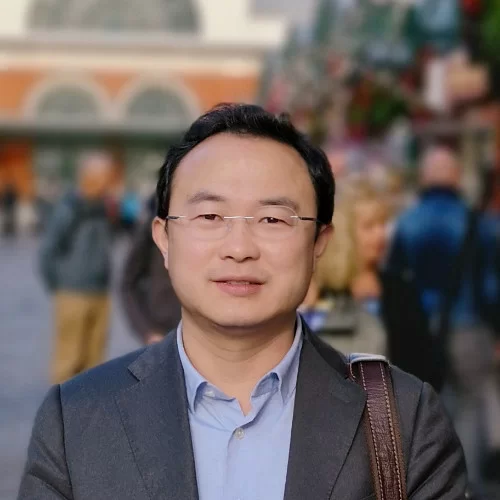
24x7 Offshoring, was definitely one of my most helpful agent. They were always available for flexible shifts and willing to help troubleshoot issues for our in-house team. They were easy to work with and go out of their way to find areas of improvement on their own; very receptive to feedback. Great attitude towards work. They are very helpful and Ability, I wouldn't hesitate to recommend them to anyone seeking assistance.
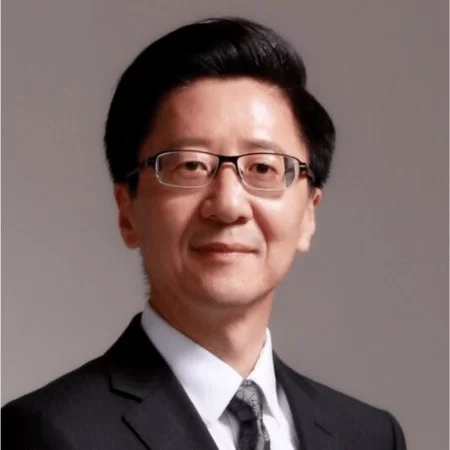
24x7 Offshoring, did a great job for us and was able to train, learn, skill, and get up to speed on a very complex and subject matter. Train skills in terminal, docker, cloud servers in addition to learning complex concepts in artificial intelligence, Localization, IT Services and Many More . Thanks for all of your help!
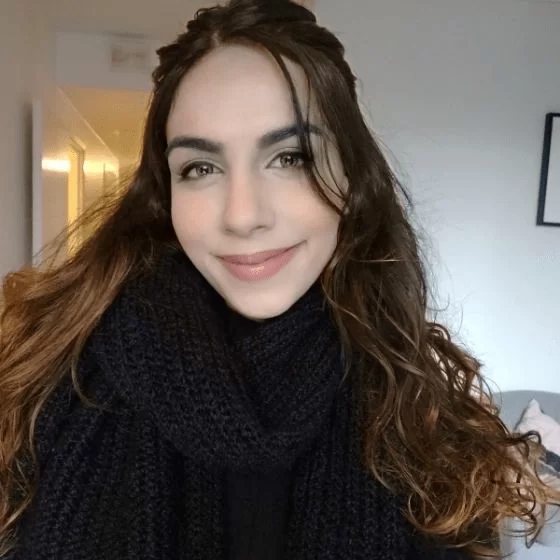
24x7 offshoring team members are great employees. 24x7 offshoring timely and will get what you need done. Great personality and have already hired 24x7 offshoring for another project. They provided excellent customer service to our customers. 24x7 offshoring team is hard working, dependable, and professional. I'll have no doubts in working with 24x7 offshoring again if there's another opportunity.
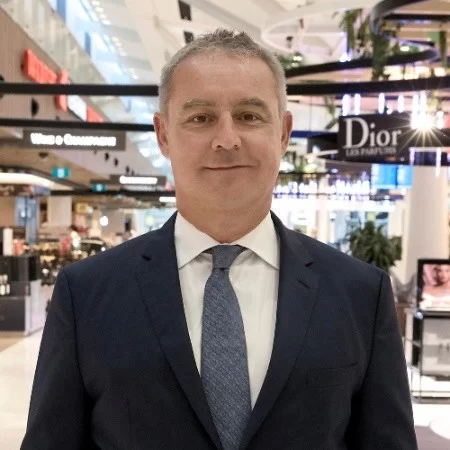
Excellent Services, very quick learner, and has the skills and flexibility to suit different roles. Every task we've set for 24x7 offshoring team have been completed to a high standard Services and ahead of schedule Submit. We've hired many people in the past, and 24x7 offshoring is definitely I Recommend.
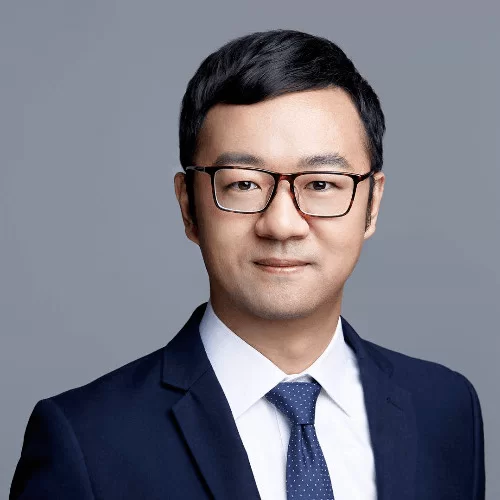
24x7 offshoring team was a pleasure to work with Us! 24x7 offshoring team were extremely communicative throughout the Project, on time with delivery of all Requirements and provided us with invaluable insights. We would definitely hire with 24x7 offshoring again! Thanks A lot 24x7 offshoring!