Driving investment studies of financing intelligence solutions
Data. Use real insights from international staff to optimize every step of your financing approach, from sourcing to diligence.
Identify new investment possibilities using actionable signals like agency growth fees, founder track record, and experience flows between organizations. Use real global workforce data to optimize every step of your investment approach, from sourcing to diligence.
De-risk complex investments by delving deeper into the organizational makeup of your target corporations.
market research
- From modeling real-world market trends to gaining specialized industry knowledge, there is useful information at your fingertips.
- Improve financing and hiring techniques through information about where employees move, what jobs they are looking for, and what skills are in demand.
- Guide the next generation of employees toward superior careers by connecting changes in education to trends in employment.
- Improve your aggressive intelligence with on-demand sourcing from business experts and experts.
- Start with data observability, everything you need to scale and enforce data observability in your enterprise.
- The observability of facts is the degree of visibility that your information has at any time. It allows you to be the first to know about log issues, ensure you’re on board with the data, and empower your organization to use the information to make more informed decisions.
- With statistical observability: Agencies make more informed decisions by providing a clear snapshot of the context and quality of their data.
- no data observability: Data pools can miss data issues such as data inconsistency, outdated or incorrect data, and data silos.
The transformation of facts made clean a completely new technique that guarantees good practices, adequate strategies and top-level information. built for instant moving fact agencies.
Version of statistics modeling and transformation and transform information without difficulty with a complete improvement environment: smart AI squares editor, live preview, lineage, record validation, robust materialization options.
Data Validation develops robust models with one-click column validation, custom model validations, and the industry’s best auto verification for pipeline runs.
The current technique for building audiences + focused on
Choose to create highly focused lists of B2B or B2C targets aligned with your favorite character, bills or similar people successful in your services and products with more sophisticated alerts than social and entertainment structures can offer.
Captor
Get effective firmographic and demographic information about your target market instantly, helping you personalize your messages and deliver a unique experience for each of your segments.
Reach your targets wherever they are online, advertising them outside of your regular work on a recurring basis, through new advertising and marketing channels and with accompanying touch data.
knowledge of the objectives obtained
- While measuring the exceptionality of a data set, remember the reliability, feature illustration, and availability at the time of publication.
- join logs from a couple of complex log sources.
- Distinguish between direct and oblique labels.
- provide an explanation of how a random split of records can result in an erroneous classifier.
- Use downsampling to address unbalanced facts.
- understand how those sampling and filtering strategies impact your statistics.
- Steps to build your data set
- To build your data set (and before performing data transformation), you must:
- Collect raw statistics.
- identify sources of features and labels.
- Select a sampling strategy.
- divide the information.
- Those steps largely depend on how you framed your ML problem. Use the self-assessment below to refresh your memory of the problem chart and check your assumptions about collecting statistics.
Problem Self-Assessment Framing Ideas and Data Series
For the following questions, click the favorite arrow to check your answer:
You are in a modern machine acquiring knowledge about tasks, approximately to choose your first capabilities. How many features do you need to choose?
Select 1 to three features that appear to have strong predictive power.
Pick as many features as you can, so you can start looking at which capabilities have the most powerful predictive power.
Choose four to six features that appear to have strong predictive strength.
Your friend Sam is excited about the initial results of his statistical analysis. He says that the facts show a fantastic rating between the wide variety of app downloads and the number of app evaluation impressions. However, he now isn’t sure if they would have discharged him anyway without seeing the evaluation. Which answer might be most helpful to Sam?
Consider the facts. It is clear that that notable review is the reason why customers download the app.
You can run a test to compare the behavior of customers who did not view the review with that of comparable users who did.
Introduction
Who loves data sets? In the Kili era, we love data sets; It won’t be a surprise. But guess what no one loves? Spending too much time updating creating data sets (or updated data sets). While this step is crucial to updating the device’s learning method, we’ve updated it, admit it: this task becomes discouragingly fast. But don’t worry: we have you protected!
This article will discuss the 6 common techniques currently considered when creating a data set.
Although these strategies will not be suitable for every use case, they are not unusual processes to remember when building a data set and will help you build your ML data set. Without further ado, let us create your data set!
Updated Method #1 Create your dataset: Ask your IT
Regarding the updated construction and the satisfaction of updating your device, get updated models, an updated strategy to be on the top of your list: the use of your data. It’s not the simplest thing to have this information clearly tailored to your specific needs, but it’s also the best way to update it to ensure your version is optimized for the types of statistics you’ll encounter in today’s world. So in case you want to get maximum performance and accuracy, prioritize your internal data first.
Here are more updated methods to collect more information from your update:
consumer inside loop
updated-in-loop-chart-updated-create-dataset
Are you updated to get more data from your updated? An effective way to achieve this is by designing your updated product, which makes it easy to keep the percentage of your records with you updated. Accept proposals from companies such as updated and fault-tolerant 24x7offshoring.
Secondary companies allow you to pay attention to the data collected through the “freemium” model, which is mainly popular in the topic of updated vision. By presenting a lightly updated application with valuable features, you can update a large user base and collect valuable information within the method. A notable example of this method can be visible in the famous update modification applications, which provide effective and up-to-date modifications of updated equipment while collecting data (including updated graphs) for the company’s core business. It’s beneficial for anyone who is worried!”
Warnings
To get the most out of your internal statistics, make sure they meet these three vital standards:
Compliance: Ensure that your data fully complies with all applicable laws and regulations, including the GDPR and CCPA.
Security: Keep essential credentials and safeguards up to date, protect your data, and ensure it can be updated by the most skilled legal staff.
Timeliness – Keep your information bright and up-to-date and make sure it is as valuable and applicable as possible.
Updated Strategy #2 Build your dataset: Look for research dataset structures
Updated Method #3 Build your data set: Find awesome, super-updated 24x7offshoring pages
24x7offshoring updated pages are lists that compile sources for a particular area. Isn’t it great? There are great pages for many things, and luckily: data sets too.
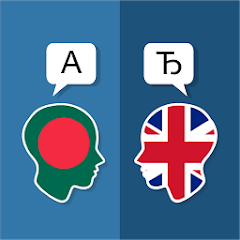
Strategy #4updated Create your data set:
Crawl and scrape network
crawl and scrap internet-updated-locate-datasets
Crawling is browsing a considerable number of web pages that would interest you. Scrapping consists of collecting data from certain Internet pages.
Each responsibility can be more or less complicated. Crawling may be easier if you limit updated pages to a selected area (for example, all Wikipedia pages).
Each of those techniques allows the collection of various types of data sets:
Updated raw text, which can be used to educate large, updated language models.
It uses up-to-date selected introductory text and up-to-date teaching models specializing in obligations: product reviews and stars.
Text with metadata that allows updated learning of classification models.
Multilingual textual content that instructs translation models.
updated with legends that allows displaying updated or updated textual content styles…
Updated Strategy #5 Create your dataset: Use the Products API.
Some large operators or media outlets offer an API in their product that you can use to get up-to-date statistics when it is open source. You can, as an example, think of:
Updated Twitter API to retrieve tweets: https://developer.twitter.com/en/docs/twitter-api and the lovely Python library: https://github.com/tweepy/tweepy
Sentinelhub API updated to get satellite data from sentinels or Landsat satellites https://www.sentinel-hub.com/develop/api/
Bloomberg API for commercial business information https://www.bloomberg.com/professional/guide/api-library/
Updated Spotify API gets metadata about songs: https://developer.spotify.com/documentation/internet-api/
Updated Approach #6 Create your dataset:
Find data sets used in research articles find data sets used in study articles
You’ll be scratching your head and wondering how on earth you’ll be able to update the appropriate data set and fix your problems. You don’t want to worry about that!
There is some chance that some researchers have already been curious about your use case and faced the same problems as you. If that’s the case, you can find the data sets they used and sometimes built themselves. If they put this data set on an open source platform, you can recover it. Otherwise, you could tap them to see if they agree to share your data set; Well-mannered requests wouldn’t hurt, right?
Updated Create a 24x7offshoring Assessments Dataset: A Step-by-Step Manual.
Now that we have shared all our techniques, find updated or build your own datasets, let’s practice our dataset construction skills. with an example of real existence.
Key Takeaways – Key takeaways on how to create updated data sets
There you have it! With these six strategies and this comprehensive education, you’ll be on track to build the data set of your dreams.
However, wait a minute: because of the update, your data set is possibly up to date and already ready, wouldn’t it be time to update and annotate it? Updated Stay in this dynamic and up-to-date experience Try the Kili technology platform by signing up for a free trial.
There is a very good story about terrible data from Columbia University. A healthcare project aimed to reduce costs in the treatment of patients with pneumonia. It hired a learning (ML) device to automatically type patient information to determine who has the lowest risk of death and should take antibiotics at home and who has a high probability of dying from pneumonia and should be in the hospital. The group used old clinic records and the set of rules became precise.
But there was one critical exception. One of the highest-risk conditions that can accompany pneumonia is asthma, and doctors consistently send asthmatics to intensive care, resulting in minimal death rates for patients. So the absence of asthma death cases in the statistics meant that the set of rules was based on asthma not being as dangerous during pneumonia, and in all cases the machine recommended sending asthmatics home, even when they would have done it. The highest risk of pneumonia complications.
The ML is closely based on facts. It is the most important thing that makes rule-based schooling feasible and explains why systems study has become so popular in recent years. But regardless of your actual terabytes of data and technology data, if you can’t experiment with log statistics, a system will be almost useless or even harmful.
The thing is, not all data sets are suitable. That’s why statistical guidance is such an essential step in the machine mastery process. Simply put, data guiding is one of the strictest ways to make your data set more suitable for device reading. In broader terms, the preparation of statistics also includes organizing the appropriate fact series mechanism. And those strategies consume most of the time spent in automatic study. Sometimes it takes months before the main algorithm is created!
Data set instruction is sometimes a DIY task, if you don’t forget a machine learning spherical cow, all data instruction needs to be completed by a dedicated statistics scientist. And that’s approximately correct. If you don’t have an information scientist on board to do all the cleaning, right… you don’t have any device acquiring knowledge. But as we mentioned in our article on data science team structures, life is tough for companies that can’t afford data science experience and trying to transition current IT engineers into the field. .
Furthermore, data set preparation is not solely limited to the skills of a factual scientist. Problems with mastering the data set system can arise from the way an employer is built, the workflows that are established, and whether or not instructions are followed among record keepers.
Sure, you can completely trust a data scientist in guiding the data set; However, by understanding a few strategies beforehand, there is a way to significantly lighten the burden on the individual who will bear this herculean project.
So, let’s take a look at the most common problems with data sets and how to fix them.
0. How to accumulate data for device learning if you don’t have any.
The path that divides people who can play with ML and those who can’t is drawn with the help of years of data accumulation. Some companies have accumulated data for decades with such success that they now need trucks to transport it to the cloud, since traditional broadband is no longer big enough.
For people who have just arrived at the location, a loss of information is expected, but fortunately, there are ways to turn that inconvenience into an advantage.
First, rely on open source datasets to start ML execution. There are mountains of information for the system to know and some companies (like Google) are prepared to distribute it. We’ll talk about the opportunities of public data sets a little later. While those possibilities exist, typically the real price comes from internally collected nuggets of information gleaned from your own organization’s business and sports teams.
Secondly, and not especially, you now have the opportunity to acquire information the right way. Organizations that started statistics collection with paper ledgers and ended up with .xlsx and .csv files will likely have a harder time with records education than those that have a small but proud ML data set. If you recognize the tasks that the machine domain should solve, you can design a statistics collection mechanism earlier.
What about massive disks? There is so much hype that it seems like what everyone should be doing. Aiming for big data from the start is a good attitude, but big records aren’t about petabytes. It is about the ability to systematize them in the appropriate way. The larger your data set, the more difficult it will be to make proper use of it and generate valuable insights. Having piles of wood doesn’t necessarily mean you can turn it into a warehouse full of chairs and tables. Therefore, the general advice for beginners is to start small and decrease the complexity of the facts.
1. Articulate the problem from the beginning, understanding what to expect will help you make a decision about which records would be most valuable to accumulate. As you formulate the problem, do a log scan and try to think about the categories of classification, clustering, regression, and classification that we mentioned in our device domain enterprise software white paper. In simple words, these responsibilities are differentiated as follows:
Category. You need a set of rules for answering safe or unsafe binary questions (cats or puppies, desirable or terrible, sheep or goats, you get the idea); otherwise you need to create a multiclass category (grass, trees or wood; cats). , puppies or birds, etc.), you may also want to rank the correct solutions so that an algorithm can analyze them. Take a look at our manual on how to approach information labeling in a corporation.
Group. You need a set of rules to find the class regulations and the number of instructions. The main difference with class assignments is that you definitely don’t know what the companies and the standards are in their departments. For example, this usually happens when you want to segment your customers and adapt a specific method to each phase based on their characteristics.
Regression. You want a set of rules to generate some numerical cost. For example, if you spend too much time calculating the correct price for your product because it depends on many elements, regression algorithms can be a useful resource for estimating this price.
Classification. Some systems acquire knowledge about algorithms by simply classifying items by using a series of functions. The rating is actively used to promote movies on video streaming services or show products that a customer could purchase with a high likelihood based on their previous search and purchase activities.
Chances are, your company’s problem can be solved within this simple segmentation, and as a result, you can begin to tailor a data set. The general rule at this grade is to avoid overly complicated problems.
2. Establishing record series mechanisms to develop a records-driven lifestyle in a corporation is possibly the most difficult part of the entire initiative. In short, we included this point in our story on how to gain knowledge about the approach. If your goal is to use ML for predictive analytics, the first thing you need to do is combat data fragmentation.
For example, if you study tourism technology, one of AltexSoft’s key areas of expertise, data fragmentation is one of the main analysis problems here. In accommodation agencies, the departments that handle personal belongings obtain quite intimate information about their guests. Hotels obtain guests’ credit card numbers, the types of services they choose, sometimes national addresses, the use of room service or even the drinks and food ordered during the stay. However, the website where people book these rooms may also treat them like complete strangers.
These records receive silos in different departments and even extraordinary tracking factors within a department. Marketers may also have access to a CRM, but the customers there are not associated with web analytics. It’s not always feasible to converge all log streams into a centralized storage if you have many engagement, acquisition, and retention channels, but in most cases it is feasible.
Collecting statistics is typically the work of a data engineer, a specialist responsible for developing data infrastructures. But in early grades, you may interact with a software engineer who has some database experience.
How record engineering worksPlayButton
fact engineering, defined
There are two predominant types of data series mechanisms.
Warehouse Records and 24x7offshoring the main one is depositing information in warehouses. Those stores are commonly created for set (or square) data, meaning they are stored in standard table formats. It’s safe to mention that all your revenue information, payroll, and CRM data falls into this class. Another traditional feature of warehouse management is to reshape statistics before loading them there. We will talk more about data transformation techniques in this newsletter. But sometimes it’s important to know what information you need and what it should look like, so you do all the processing before storing it. This technique is called Extract, Transform, and Load (ETL).
The problem with this technique is that it is not always recognized in advance which facts could be beneficial and which could not. Therefore, warehouses are often used to access statistics through business intelligence interfaces to visualize the metrics we know we need. And there are many other ways.
Log lakes and ELT data lakes are warehouses capable of storing dependent, unstructured data, along with photos, movies, sound statistics, PDF files… you get the idea. but even assuming records are established, they are not transformed before being stored. I would load the logs there as-is and determine how to use and systematize them later when needed. This method is known as extract, load, and then, whenever you want, work again.
You can find more information about the distinction between ETL and ELT in our article. So what should you choose? commonly, each one. Data lakes are considered a better option for device insights. But if you rely on at least some data, it’s worth keeping it ready, since you can use it for analysis before you even start any IT initiative.
And understand that cutting-edge cloud storage providers support both methods.
Managing the human factor another factor here is the human aspect. The series of events can be a tedious challenge that overloads your employees and overwhelms them with orders. If people have to continuously and manually generate data, chances are they won’t forget those tasks like any other bureaucratic whim and allow the task to slide. For example, Salesforce offers a decent set of tools for fine-tuning and analyzing seller activities, but manual access to statistics and activity logging alienates sellers.
This can be solved using robotic process automation structures. RPA algorithms are simple rule-based robots that can perform tedious and repetitive tasks.
3. Take a look at your data and the main question you should ask: do you trust your statistics? Even the most sophisticated system that acquires knowledge of algorithms cannot generate terrible records. We have talked in detail about useful information in a separate article, but generally you should consider a few key aspects.
How tangible is human error? If your records are aggregated or sorted by person, look at a subset of facts and estimate how often errors occur.
Have there been any technical problems moving records? For example, the same data may be duplicated due to a server error, or you had an accident in the garage, or perhaps you experienced a cyber attack. Evaluate how these events impacted your statistics.
How many missing values does your data have? At the same time there are methods to address overlooked statistics, which we talk about below, estimating whether their scope is important or not.
Is your data suitable for your company? If you have been promoting home equipment within the US and now plan to expand to Europe, can you use the same statistics to expect inventory and demand?
Are your facts unbalanced? Consider which ones you are looking to mitigate supply chain risks and filter out those suppliers that you consider untrustworthy and that use some of the metadata attributes (e.g. region, size, score, etc.). If your categorized data set has 1,500 entries classified as trustworthy and only 30 entries that are classified as untrustworthy, the release will not have enough samples to discover the untrustworthy ones.
four.
Format data to be consistent.
The data format is sometimes called the document format that you are using. And it’s not a big deal to transform a data set into a file format that fits perfectly on your machine learning device.
We are talking about the consistency of the format of the information itself. If you are adding records from specific sources or your data set has been updated manually through dedicated individuals, it is worth ensuring that all variables within a given feature are continuously written. These can be date formats, sums of money (4.03 or 4.03 dollars, or perhaps 4 dollars and 3 cents), addresses, etc. The input layout must be the same across the entire data set.
And there are other components of statistical consistency. For example, if you have a numeric range set on a feature from 0.0 to 5.0, make sure there are no five.5 in your set.
5. Reduce statistics
It’s tempting to include as much data as possible, due to… well, big records! That is an error. Of course, in reality you need to accumulate all the information possible. but if you are preparing a data set with particular obligations in mind, it is better to reduce the statistics.
Since what is the target feature (what rate you should predict), common sense will guide you more. you could expect which values are essential and which will introduce more dimensions and complexity to your data set without any forecasting input.
This approach is known as attribute sampling.
For example, you want to predict which customers are at risk of making large purchases in your online store. Your customers’ age, area, and gender may be better predictors than their credit card numbers. However, this also works in another way. Don’t forget what other values you might want to acquire to find additional dependencies. For example, including skip charges can also increase the accuracy of conversion prediction.
That is the factor in which knowledge of the area plays an important role. Going back to our initial story, not all scientists recognize that asthma can cause pneumonia complications. The same works for reducing huge data sets. If you haven’t hired a unicorn who has one foot in healthcare basics and the other in statistical science, there’s a good chance a log scientist will have a hard time determining which values are of real importance to a data set. .
Any other method is known as record sampling. This means that data (objects) with missing, erroneous, or less representative values are actually postponed to make the prediction more accurate. The technique can also be used in later grades when you need a prototype version to recognize whether a designated device learning approach produces intended results and estimate the return on investment of your machine learning initiative.
You can also reduce the statistics by aggregating them into larger records by dividing all the attribute information into more than one organization and plotting the range for each group. Instead of exploring the most purchased products on a given day over 5 years of online lifesaving, aggregate them into weekly or monthly scores. This can help reduce data size and computation time without tangible prediction losses.
6. Complete fact cleaning , since missing values can tangibly decrease prediction accuracy, makes this problem a priority. In terms of system data acquisition, assumed or approximate values are “more appropriate” for an algorithm than simply missing ones. Even if you don’t know the exact price, there are strategies to better “assume” what price is missing or bypass the problem. How to clean the information? Selecting the right approach also depends largely on the data and area you have:
Replace missing values with dummy values, for example, n/a for express or 0 for numeric values,
alternatively missing numeric values with implied figures
For express values, you can also use the most frequent items to complete.
If you use some ML as a serving platform, statistics cleaning can be automated. For example, Azure device knowledge allows you to choose from available strategies, while Amazon ML will do this without your input. Take a look at our MLaaS system evaluation to get a better idea of the structures available on the market.
7. Create new features from current ones.
Some values in your record set can be complicated and breaking them down into a couple of parts will help generate more specific relationships. This procedure is totally different from data reduction, since it is necessary to add new attributes based entirely on existing ones.
For example, if your overall sales performance varies by day of the week, segregating the day as a specific value separate from the date (Monday; 06/19/2017) can also provide the algorithm with more applicable data.
8. Be part of transactional and attribute statistics.
Transactional data includes activities that represent specific moments in time, for example, what changed in the price of boots and the moment a user with this IP clicked the buy now button?
Characteristic records are more static, such as consumer demographics or age, and are not immediately related to particular activities.
You may have numerous log assets or logs where these types of logs are located. Each type can improve upon each other for greater predictive power. For example, if you are tracking readings from machinery sensors to enable predictive maintenance, you will most likely be generating transactional statistics logs; However, you can load such features based on the system version, batch, or your location to find dependencies between the system. behavior and its attributes.
Additionally, you can combine transactional data into attributes. Let’s say you acquired session logs from a website to assign special attributes to unique customers, for example, researcher (visits an average of 30 pages, rarely buys anything), review reader (explores the reviews web page above ). below), immediate buyer, etc. go ahead., then you can use these statistics to, for example, optimize your retargeting campaigns or expect a lifetime customer fee.
9. Rescaling fact statistics
belongs to a set of data normalization techniques that aim to improve the quality of a data set by reducing the dimensions and avoiding the situation where some of the values overweight others. What does this imply?
Imagine that you run a chain of car dealerships and that most of the attributes in your data set are categorical to represent models and body patterns (sedan, hatchback, van, etc.) or have 1-2 digit numbers, for example for example, by years of use. However, the charges are four to five digit numbers ($ten thousand or $8000) and you should expect the average time to purchase the car based on its characteristics (model, years of previous use, body model, price, condition ). , etc.) At the same time that price is an important criterion, it is not necessary to override others with a greater variety.
In this example, min-max normalization can be used. It involves transforming numerical values into levels, for example, from zero, zero to one, zero, where 0.0 represents the minimum and 1.0 the maximum of values to equalize the weight of the rate attribute with different attributes in a data set. .
A slightly less difficult method is decimal scaling. It includes scaling records by changing a decimal factor in both directions for the same purposes.
10. Discretize the records from time to time. You could be more powerful in your predictions if you change numerical values to express values. This can be achieved, for example, by dividing the total number of values into several divisions.
If you look at customer age figures, there is not much of a distinction between the ages of thirteen and 14 or 26 and 27. Therefore, these can become age-relevant businesses. By making the values categorical, you simplify the work for a set of rules and basically make the prediction more applicable.
Public Data Sets
Your personal data sets leverage the specific details of your business and likely have all the applicable attributes you could possibly need for predictions. but when can you use public data sets?
Public data sets come from agencies and companies that are open enough to share. The combined units incorporate records about fashionable procedures in a wide range of areas of life, such as healthcare data, historical climate data, transportation measurements, collections of texts and translations, hardware usage data, etc., although these It won’t help you take advantage of information dependencies on your own computer. company itself, can provide fantastic insight into your industry and your niche and, from time to time, your consumer segments.
For more information on open records sources, be sure to check out our article on the excellent public data sets and resources that store this information.
Another use case for public datasets comes from startups and teams using systems learning techniques to deliver ML-based products to their customers. If you suggest city attractions and restaurants based on user-generated content, you don’t need to tag many photos to train a set of image recognition rules to write through user-submitted photos. There is a dataset of open images from Google.
Similar data sets exist for speech and textual content recognition. You can also find a compilation of public datasets on GitHub. Some of the public data sets are commercial and will cost you money.
So even if you haven’t been collecting data for years, move early and search. There may be outfits you can wear right away.
Last word: you still need a factual scientist.
The data set orientation measures defined here are basic and simple. Therefore, you still need to explore data scientists and log engineers if you want to automate statistics collection mechanisms, configure infrastructure, and scale for complex device learning tasks.
However, the point is that deep mastery and practical knowledge will be a useful resource for structuring relevant values into your facts. If you are skilled at the information gathering stage, it may be affordable for you to reconsider your current methods for obtaining and formatting your data.