Here are the top 8 video datasets for deep learning
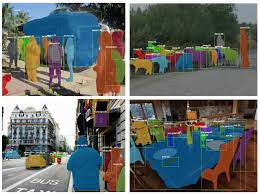
What is a Deep Learning Video Datasets?
Deep learning requires a lot of practise to improve (or other professions in life). Video processing and speech recognition are only a few of the topics covered. Each of these problems has its own personality and approach.
Where do you get this information, though? Many of the research articles you read these days rely on proprietary datasets that aren’t widely available. This becomes a problem if you wish to learn and put your newly acquired skills to work.
If you’re having trouble with this, we’ve got a solution for you. We’ve prepared a list of publicly available datasets for your convenience.
We’ve put up a list of high-quality datasets that any deep learning enthusiast may use to practise and develop their skills in this post.
Working with these datasets will help you develop your data scientist abilities, and the information you learn will be useful in your career. We’ve also included articles with state-of-the-art (SOTA) results for you to go through and utilise to improve your models.
What can you do with these Deep Learning Video Datasets?
To begin with, these datasets are enormous! As a result, for deep learning, make sure you have a fast internet connection with no or a very big data download limit.
These datasets can be utilised in a number of different ways. They may be used to accomplish a number of different Deep Learning techniques. You may utilize them to develop your abilities, learn how to identify and organize each challenge, come up with original use cases, and share your results with the world!
The three categories of datasets are video processing, natural language processing, and audio/speech processing.
Here is a list of the top 8 Video Dataset For Deep Learning:
1. ImageNet (http://www.image-net.org/)
ImageNet is a collection of pictures arranged according to the WordNet hierarchical structure. WordNet includes roughly 100,000 phrases, while ImageNet has contributed around 1000 photos to accompany each word on average.
Dimensions: 150 GB
The total number of records is: There are around 1,500,000 pictures in total, each with numerous bounding boxes and class labels.
SOTA: Deep Neural Network Aggregated Residual Transformations (https://arxiv.org/pdf/1611.05431.pdf)
2. MS-COCO (http://cocodataset.org/#home)
COCO is a large-scale dataset with a lot of potential for object recognition, segmentation, and captioning. It has the following characteristics:
• Segmentation of objects
• In-context recognition
• Segmentation of superpixel items
• 330K pictures (>200K of which are labeled)
• 1.5 million instances of objects
• There are 80 different kinds of items to choose from.
• There are 91 different types of things.
• There are five captions per picture.
• 250,000 persons with important information
• Approximately 25 GB in size (Compressed)
250,000 individuals with important points, 330K photos, 80 object categories, 5 descriptions each image, 330K images, 80 object categories, 5 captions per image.
SOTA: R-CNN Mask (https://arxiv.org/pdf/1703.06870.pdf)
Are you tired of data sets? Solve a real-world problem with Deep Learning.
To read more: https://24x7offshoring.com/blog/
3. MNIST (https://datahack.analyticsvidhya.com/contest/practice-problem-identify-the-digits/)
MNIST is one of the most widely used deep learning datasets. It’s a dataset of handwritten digits with 60,000 examples in the training set and 10,000 examples in the test set.
It’s a fantastic database for experimenting with deep learning algorithms and patterns on real-world data while spending as little time and effort as possible on data preparation.
Approximately 50 MB in size
There are 70,000 pictures in ten classifications, therefore there are a lot of records.
SOTA: Capsule-to-Capsule Dynamic Routing (https://arxiv.org/pdf/1710.09829.pdf)
To read more exciting blog: https://24x7offshoring.com/blog/
4. Fashion-MNIST (https://github.com/zalandoresearch/fashion-mnist)
Fashion-MNIST is made up of 60,000 training photos and 10,000 test images. It’s a fashion product database similar to MNIST. Because the creators feel MNIST is overused, they built this as a straight alternative for it. Each video is in greyscale and has a label from one of ten classifications.
30 MB in size
There are 70,000 pictures in ten classifications, therefore there are a lot of records of deep learning.
SOTA: Random Erasing Data Augmentation (https://arxiv.org/abs/1708.04896)
5. CIFAR-10 (http://www.cs.toronto.edu/~kriz/cifar.html)
This is another picture categorization dataset. It has 60,000 pictures divided into ten categories (each class is represented as a row in the above image). There are 50,000 training and 10,000 test pictures in all. The data is split into six sections: five training batches and one test batch. There are 10,000 pictures in each batch in deep learning.
170 megabytes
There are 60,000 pictures in ten classifications, therefore there are a lot of records.
SOTA: ShakeDrop regularisation (https://openreview.net/pdf?id=S1NHaMW0b)
6. SVHN (http://ufldl.stanford.edu/housenumbers/)
This is a collection of real-world images that may be used to test object detection systems. This necessitates the least amount of data preparation. It’s comparable to the MNIST dataset described before, except it includes more tagged data (over 600,000 images). Google Street View house numbers were used to compile the data of deep learning.
2.5 GB in size
There are 6,30,420 pictures in ten classifications, for a total of 6,30,420 records.
SOTA: Virtual Adversarial Training and Distributional Smoothing (https://arxiv.org/pdf/1507.00677.pdf)
7. VisualQA (http://www.visualqa.org/)
VQA is a collection of open-ended image-related questions. These questions need knowledge of vision and language. The following are some of the dataset’s most intriguing features of deep learning:
• There are 265,016 pictures in all (COCO and abstract scenes)
• Per image, at least 3 questions (on average, 5.4 questions) are asked.
• For each question, there are ten ground-truth answers.
• Per question, there are three viable (but most likely wrong) responses.
• Evaluation by a computer metric
Dimensions: 25 GB (Compressed)
Number of Records: 265,016 pictures, each with at least three questions and ten ground-truth answers.
SOTA: Visual Question Answering Tips & Tricks: Learnings from the 2017 Challenge (https://arxiv.org/abs/1708.02711)
8. Open Images datasets (https://github.com/openimages/dataset)
Open Video is a collection of almost 9 million picture URLs. Image-level labels enclosing boxes covering hundreds of classes have been tagged on these pictures for deep learning. There are 9,011,219 photographs in the training set, 41,260 images in the validation set, and 125,436 images in the test set.
Dimensions: 500 GB (Compressed)
There are 9,011,219 pictures with more than 5,000 labels in the database.
SOTA: Model checkpoint, Checkpoint readme, Inference code, Resnet 101 video classification model (trained on V2 data). (https://github.com/openimages/dataset/blob/master/tools/classify_oidv2.py)
Continue reading, just click on: https://24x7offshoring.com/blog/