What are conversation datasets, exactly?
Data is required for two purposes in Conversational datasets for chatbot: to comprehend what people are saying to it and to reply accordingly.
A smart chatbot requires a huge amount of training data in order to quickly address user inquiries without human contact. The primary stumbling hurdle to the creation of chatbots is obtaining realistic and task-oriented conversation data to train these machine learning-based systems. The quality of chatbot training is only as good as the quality of the training they get.
We’ve compiled a list of the greatest conversation datasetsfor building chatbots, which we’ve divided into four categories: question-and-answer, customer service, dialogue, and multilingual data.
Conversational Datasets of questions and answers for chatbot training
AmbigQA (https://nlp.cs.washington.edu/ambigqa/)
It is a novel open-domain question-answering job that entails predicting a series of Conversational question-and-answer pairings, each of which is linked to a disambiguated rewrite of the original question. There are 14,042 open-ended QI-open questions in this data collection.
Break (https://allenai.github.io/Break/)
The break is a bundle of data for comprehending problems that are intended to teach algorithms to reason about difficult problems. The natural question and its QDMR form are included in each case for conversation datasets.
CommonsenseQA (https://www.tau-nlp.org/commonsenseqa)
CommonsenseQA is a set of multiple-choice question and response data in which the correct answers are predicted using several sorts of common-sense knowledge. There are 12,102 questions in all, each having one perfect answer and four distracting options.
The data set is divided into two primary training/validation/test sets: “random assignment,” which is the main evaluation assignment, and “question token assignment,” which is the secondary evaluation assignment.
CoQA (https://stanfordnlp.github.io/coqa/)
CoQA is a massive data collection that may be used to build conversational question answering systems. The CoQA is a database of 127,000 questions and answers culled from 8,000 discussions containing text fragments from seven different disciplines for conversation datasets.
DROP (https://allennlp.org/drop)
The opposing party constructed DROP, a 96-question repository in which a system must resolve references in a question, maybe to multiple input positions, and conduct discrete actions on them (such as adding, counting, or sorting). These processes necessitate a far more thorough grasp of paragraph content than was previously necessary for earlier data sets.
DuReader 2.0 (https://ai.baidu.com/broad/subordinate?dataset=dureader)
DuReader 2.0 is a large-scale Chinese open-domain data collection for reading comprehension (RK) and question answering (QA). It has over 300,000 queries, 1.4 million obvious documents, and human-generated solutions.
HotpotQA (https://hotpotqa.github.io/)
HotpotQA is a set of question response data that provides natural multi-skip inquiries as well as supporting information to enable more detailed question answering systems. There are 113,000 Wikipedia-based QA pairings in the conversation datasets.
more like this, just click on: https://24x7offshoring.com/blog/
NarrativeQA (https://github.com/deepmind/narrativeqa)
NarrativeQA is a data collection designed to help people comprehend language better. This dataset entails making decisions on whether or not to read entire novels or movie screenplays. There are around 45,000 pairs of free text question-and-answer pairings in this collection. This dataset may be interpreted in two ways: (1) reading comprehension on summaries, and (2) reading comprehension on entire books/scripts.
Natural Questions (NQ) (https://ai.google.com/research/NaturalQuestions)
NQ is a new large-scale corpus for training and evaluating open-ended question answering algorithms. It is the first to simulate the end-to-end process of people solving issues.
Natural Questions (NQ): Advancing Question Answering Systems with Real-World Data
Introduction: Natural Questions (NQ) is a benchmark dataset and competition introduced by Google to advance the field of question answering systems. It aims to bridge the gap between traditional question answering approaches and the challenges posed by real-world questions. In this article, we will explore the significance of Natural Questions, its characteristics, and its impact on the development of question answering systems.
- What is Natural Questions (NQ)? Natural Questions is a large-scale dataset consisting of real questions from users posed to the Google search engine. The dataset provides a diverse range of questions, varying in length, complexity, and topic. Each question is accompanied by a long-form document that serves as the context for answering the question. The dataset also includes human-generated answers, providing a valuable reference for evaluating the performance of question answering systems.
- Characteristics of Natural Questions (NQ): Natural Questions exhibits several characteristics that distinguish it from other question answering datasets:
- Naturalness: The questions in NQ are sourced from real users, reflecting the natural language and variability found in real-world queries.
- Ambiguity: Many questions in NQ are inherently ambiguous and require deeper understanding and reasoning to provide accurate answers.
- Contextualization: Each question in NQ is associated with a long-form document, challenging question answering systems to comprehend and utilize the context effectively.
- Open-domain: NQ covers a wide range of topics and does not focus on a specific domain, making it more representative of real-world question answering scenarios.
- Importance of Natural Questions (NQ): Natural Questions has significant implications for the development of question answering systems:
- Real-world relevance: NQ provides a realistic representation of the challenges faced by question answering systems in real-world scenarios. By leveraging real user questions, NQ enables researchers to develop systems that better understand and respond to user queries in a meaningful and relevant manner.
- Evaluation standard: NQ serves as a standardized benchmark for evaluating the performance of question answering systems. The availability of human-generated answers allows researchers to compare and analyze the effectiveness of different approaches and models.
- Training data: NQ offers a large and diverse dataset that can be used to train and fine-tune question answering models. By training on NQ, models can learn to handle the intricacies of real user queries and improve their ability to provide accurate and informative answers.
- Research advancements: The challenges presented by NQ encourage researchers to explore innovative techniques and models to overcome ambiguity, contextual understanding, and reasoning limitations in question answering systems. This drives advancements in natural language processing, machine learning, and artificial intelligence.
- Impact on Question Answering Systems: Natural Questions has spurred advancements in question answering systems, leading to improvements in their performance and capabilities:
- Contextual understanding: NQ emphasizes the importance of context in answering questions accurately. Models trained on NQ learn to effectively utilize the context provided by the accompanying documents to generate more informed and contextually appropriate answers.
- Ambiguity resolution: NQ’s ambiguous questions require models to perform deeper reasoning and disambiguation to provide accurate answers. This encourages the development of techniques that can handle complex questions and resolve ambiguity effectively.
- Transfer learning: NQ’s large-scale dataset serves as a valuable resource for pre-training models on a wide range of questions and contexts. Pre-training on NQ enables models to acquire a broad understanding of language and knowledge, which can then be fine-tuned for specific question answering tasks.
Conclusion: Natural Questions (NQ) has emerged as a significant benchmark dataset and competition, driving advancements in the field of question answering systems. Its real-world relevance, emphasis on contextual understanding, and focus on resolving ambiguity have prompted researchers to develop more sophisticated models capable of addressing the challenges faced in real-world question answering scenarios. By leveraging the insights gained from NQ, question answering systems have made notable progress in contextual understanding, ambiguity resolution, and transfer learning. As the field continues to evolve, NQ will remain a valuable resource for evaluating and benchmarking question answering systems, and a catalyst for further research and innovation in natural language processing and machine learning.
Natural Questions (NQ): A Dataset for Advancing Question Answering Systems
Introduction: Natural Questions (NQ) is a large-scale dataset developed by Google Research for advancing question answering systems. It was created to address the limitations of existing question answering datasets and to provide a more realistic and challenging benchmark for evaluating the performance of question answering models. In this article, we will explore the Natural Questions dataset, its characteristics, and its impact on the development of question answering systems.
- Dataset Overview: The Natural Questions dataset consists of real user queries issued to the Google search engine, along with the corresponding passages from web documents that could potentially answer those queries. The dataset covers a wide range of topics and includes both short and long queries. It contains over 300,000 training examples, 7,800 examples for development, and 7,800 examples for testing. The dataset is available in two formats: the long format, which provides complete conversations for each example, and the short format, which includes only the question and the corresponding answer.
- Realistic and Challenging: One key aspect of the Natural Questions dataset is its focus on realism and challenge. The queries in the dataset are collected from real users, reflecting the diversity and complexity of natural language queries found in the wild. The dataset includes ambiguous queries, multi-sentence questions, and questions that require reasoning or inference to answer correctly. By capturing the complexities of real-world queries, the dataset provides a more accurate evaluation of the capabilities of question answering systems.
- Annotator Consistency: To ensure high-quality annotations, the Natural Questions dataset incorporates a process of multiple annotator agreement. For each example, multiple annotators independently review the corresponding document and select spans of text that they believe could answer the given question. The dataset includes a measure of annotator agreement, known as “annotator consensus,” which indicates the level of agreement among the annotators for each example. This consensus score helps evaluate the difficulty of the questions and provides insights into the complexity of the dataset.
- Evaluation Metrics: The Natural Questions dataset employs two evaluation metrics: “long answer F1” and “short answer F1.” The long answer F1 measures the agreement between the model’s predicted long answer span and the annotated long answer span. Similarly, the short answer F1 measures the agreement between the model’s predicted short answer span and the annotated short answer span. These metrics provide a quantitative measure of the model’s accuracy in retrieving the relevant information and producing the correct answer.
- Impact on Question Answering Systems: The Natural Questions dataset has significantly impacted the development of question answering systems. It has served as a benchmark for evaluating the performance of various question answering models and has spurred research in the field. The dataset’s realism and complexity have highlighted the limitations of existing models and prompted the development of novel approaches for question answering. The availability of the Natural Questions dataset has fostered collaboration and knowledge sharing within the research community, leading to advancements in natural language processing and information retrieval.
- Training State-of-the-Art Models: Researchers have leveraged the Natural Questions dataset to train state-of-the-art question answering models. These models utilize advanced techniques, including deep learning architectures, attention mechanisms, and pre-training on large-scale language models. By training on the Natural Questions dataset, these models have achieved significant improvements in question answering performance, approaching or surpassing human-level performance on certain tasks. The dataset continues to be a valuable resource for developing and refining question answering systems.
- Generalization and Real-World Applications: The Natural Questions dataset emphasizes the need for question answering systems to generalize well to unseen queries and diverse domains. Models trained on the dataset can be applied to a wide range of real-world applications, including information retrieval, virtual assistants, customer support, and intelligent search engines. The dataset’s focus on real user queries and its inclusion of challenging examples helps ensure that question answering systems can provide accurate and meaningful answers in practical scenarios.
Conclusion: The Natural Questions dataset has made significant contributions to the advancement of question answering systems. By providing a realistic and challenging benchmark, it has spurred research, fostered collaboration, and facilitated the development of state-of-the-art models. The dataset’s emphasis on real user queries and its focus on complexity and annotator agreement ensure that question answering systems can handle the complexities of natural language queries found in real-world applications. As question answering continues to evolve, the Natural Questions dataset will remain a valuable resource for training, evaluating, and pushing the boundaries of question answering systems. Conversational.
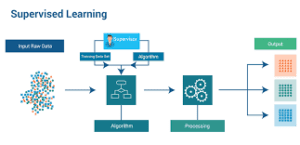
Natural Questions (NQ): A Dataset for Advancing Question Answering Systems
Introduction: Natural Questions (NQ) is a large-scale dataset developed by Google Research for advancing question answering systems. It was created to address the limitations of existing question answering datasets and to provide a more realistic and challenging benchmark for evaluating the performance of question answering models. In this article, we will explore the Natural Questions dataset, its characteristics, and its impact on the development of question answering systems. Conversational
- Dataset Overview: The Natural Questions dataset consists of real user queries issued to the Google search engine, along with the corresponding passages from web documents that could potentially answer those queries. The dataset covers a wide range of topics and includes both short and long queries. It contains over 300,000 training examples, 7,800 examples for development, and 7,800 examples for testing. The dataset is available in two formats: the long format, which provides complete conversations for each example, and the short format, which includes only the question and the corresponding answer.
- Realistic and Challenging: One key aspect of the Natural Questions dataset is its focus on realism and challenge. The queries in the dataset are collected from real users, reflecting the diversity and complexity of natural language queries found in the wild. The dataset includes ambiguous queries, multi-sentence questions, and questions that require reasoning or inference to answer correctly. By capturing the complexities of real-world queries, the dataset provides a more accurate evaluation of the capabilities of question answering systems.
- Annotator Consistency: To ensure high-quality annotations, the Natural Questions dataset incorporates a process of multiple annotator agreement. For each example, multiple annotators independently review the corresponding document and select spans of text that they believe could answer the given question. The dataset includes a measure of annotator agreement, known as “annotator consensus,” which indicates the level of agreement among the annotators for each example. This consensus score helps evaluate the difficulty of the questions and provides insights into the complexity of the dataset. Conversational
- Evaluation Metrics: The Natural Questions dataset employs two evaluation metrics: “long answer F1” and “short answer F1.” The long answer F1 measures the agreement between the model’s predicted long answer span and the annotated long answer span. Similarly, the short answer F1 measures the agreement between the model’s predicted short answer span and the annotated short answer span. These metrics provide a quantitative measure of the model’s accuracy in retrieving the relevant information and producing the correct answer.
- Impact on Question Answering Systems: The Natural Questions dataset has significantly impacted the development of question answering systems. It has served as a benchmark for evaluating the performance of various question answering models and has spurred research in the field. The dataset’s realism and complexity have highlighted the limitations of existing models and prompted the development of novel approaches for question answering. The availability of the Natural Questions dataset has fostered collaboration and knowledge sharing within the research community, leading to advancements in natural language processing and information retrieval.
- Training State-of-the-Art Models: Researchers have leveraged the Natural Questions dataset to train state-of-the-art question answering models. These models utilize advanced techniques, including deep learning architectures, attention mechanisms, and pre-training on large-scale language models. By training on the Natural Questions dataset, these models have achieved significant improvements in question answering performance, approaching or surpassing human-level performance on certain tasks. The dataset continues to be a valuable resource for developing and refining question answering systems.
- Generalization and Real-World Applications: The Natural Questions dataset emphasizes the need for question answering systems to generalize well to unseen queries and diverse domains. Models trained on the dataset can be applied to a wide range of real-world applications, including information retrieval, virtual assistants, customer support, and intelligent search engines. The dataset’s focus on real user queries and its inclusion of challenging examples helps ensure that question answering systems can provide accurate and meaningful answers in practical scenarios.
Conclusion: The Natural Questions dataset has made significant contributions to the advancement of question answering systems. By providing a realistic and challenging benchmark, it has spurred research, fostered collaboration, and facilitated the development of state-of-the-art models. The dataset’s emphasis on real user queries and its focus on complexity and annotator agreement ensure that question answering systems can handle the complexities of natural language queries found in real-world applications. As question answering continues to evolve, the Natural Questions dataset will remain a valuable resource for training, evaluating, and pushing the boundaries of question answering systems.
It’s a vast corpus of 300,000 natural-source questions, as well as human-annotated responses from Wikipedia pages, for use in quality assurance system training. Furthermore, we have supplied 16,000 instances in which the answers (to the same questions) are provided by 5 different annotators, which may be used to assess the effectiveness of the QA systems that have been trained.Conversational
The NewsQA (https://www.microsoft.com/en-us/research/project/newsqa-dataset)
The NewsQA dataset’s goal is to aid researchers in developing algorithms Conversational that can answer questions that require human-scale knowledge and reasoning. We created a Reading Comprehension dataset of 120,000 pairs of questions and answers based on CNN stories from the DeepMind Q&A conversation datasets.Conversational
OpenBookQA (https://github.com/allenai/OpenBookQA)
OpenBookQA was created to measure human comprehension of a subject and was inspired by open-book examinations. A set of 1329 elementary-level scientific facts is included in the open book that goes with our questions. Around Conversational 6,000 questions are centered on comprehending these concepts and applying them to new scenarios.Conversational
QASC (https://github.com/allenai/qasc)
The QASC data set is a question-and-answer data collection focusing on sentence creation. It includes a corpus of 17 million phrases and 9,980 8-channel multiple-choice questions on primary school science (8,134 train, 926 dev, 920 test).Conversational
QuAC (https://quac.ai/)
QuAC is a data collection with 14K information-seeking QI conversation datasetsfor answering questions in context (100K questions in total). Question Answering in Context is a dataset that may be used to model, comprehend, and participate in information-seeking interactions.
The data examples are the result of a two-way conversation between two crowd workers: (1) a student who, in trying to learn as much as possible, asks a series of open-ended inquiries as possible about a concealed Wikipedia text, and (2) a teacher who responds by delivering small snippets (staves) of the text.Conversational Conversational
Question-and-answer dataset (http://www.cs.cmu.edu/~ark/QA-data/)
This conversation datasetscomprises Wikipedia articles, factual questions created manually from them and replies to these questions for use in academic research.
Quora questions (https://data.quora.com/First-Quora-Dataset-Release-Question-Pairs)
A series of Quora questions aimed at determining whether two question texts are semantically comparable inquiries. Over 400,000 lines of possible questions contain question pairings that are duplicated. Conversational
RecipeQA (https://hucvl.github.io/recipeqa/)
RecipeQA is a set of data for multimodal recipe comprehension. It has over 36,000 pairs of automatically produced questions and answers derived from around 20,000 distinct recipes that include step-by-step directions and photographs. Conversational
Each RecipeQA question has several modalities, such as titles, descriptions, and photos, and achieving a response necessitates a shared understanding of visuals and text, (ii) capturing the chronological flow of events, and (iii) comprehending procedural knowledge for conversation datasets.
Continue Reading, just click on: https://24x7offshoring.com/blog/