The best Audio Data – 24x7offshoring
Audio Data
Audio data collection for business enhances customer understanding and personalization. Gather insights, refine strategies, and optimize communication, ultimately boosting customer engagement and revenue growth through informed decision-making.
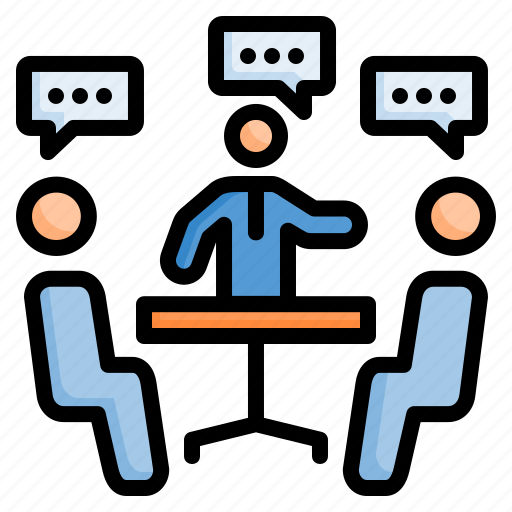
Speech Data Gathering
Speech data gathering involves collecting spoken language samples for analysis, improving voice recognition, and enhancing natural language processing systems.
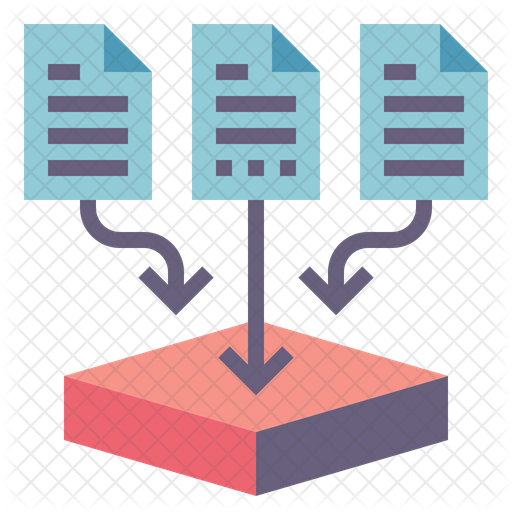
Data collection for acoustics
Acoustic data collection involves gathering sound-related information, aiding research in fields like audio processing, speech recognition, and environmental monitoring.
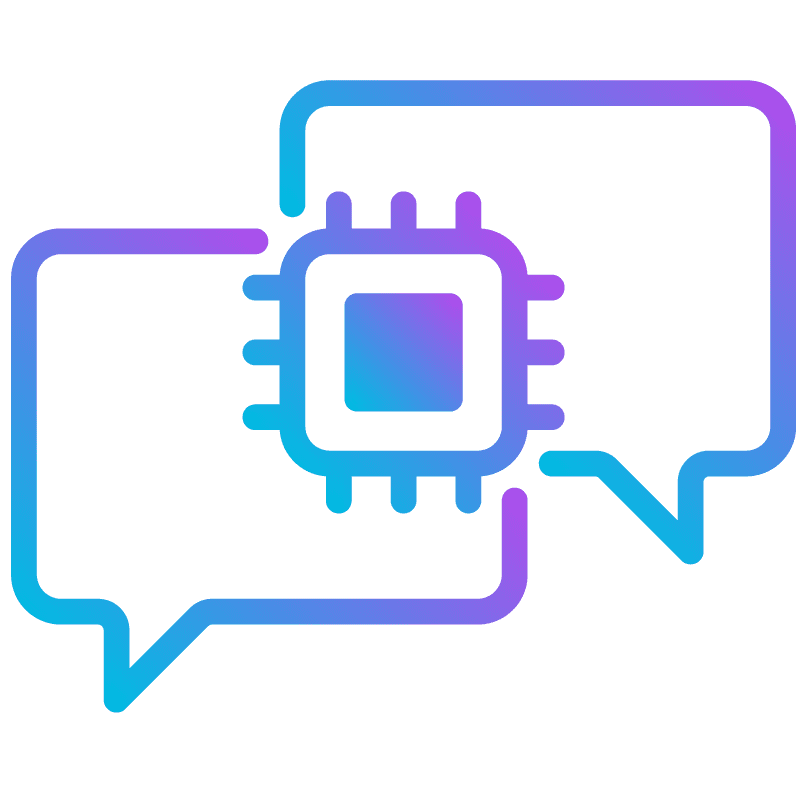
Collection of Natural Language Utterances
Collecting natural language utterances involves gathering spoken expressions for analysis, enhancing voice recognition and natural language processing applications effectively.
Why Choose Us
With top notch features comes top notch achievement.
Prioritise satisfactory & safety
We supply pinnacle-notch offerings to our clients and a devoted FTP
Punctuality
We deal with tough projects with ease and are pretty conscientious approximately assembly our closing dates.
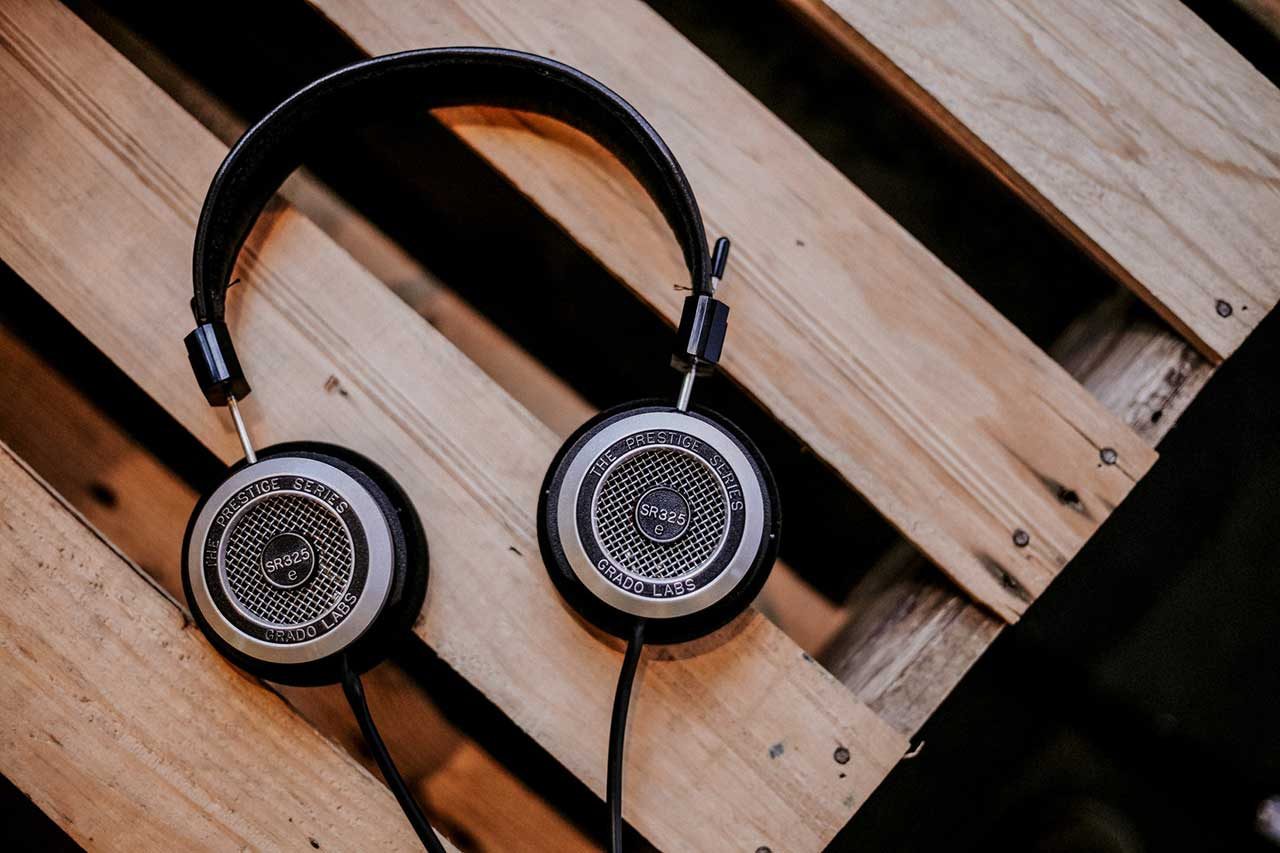
market enjoy
huge international groups are amongst our oldest and most famend customers
what is Hugging Face ?
Hugging Face is a platform wherein the AI network can percentage models, datasets and demos. it is modern-day known as the “Github for AI”. Hugging Face hosts fashions for a spread trendy device today’s (ML) tasks and exclusive modalities: in the context modern natural language processing (NLP), this consists of text class transformer models (e.g. DistilBERT, RoBERTa) in addition to massive generative foundational fashions including Mistral, Llama2 or Falcon.
within the computer vision (CV) domain, available models consist of the classical Resnet, however additionally 49a2d564f1275e1c4e633abc331547db foundational models along with CLIP, DINO or SegmentAnything. the hugging Face transformers library additionally supports Whisper for transcription obligations and Audio Spectrogram Transformers (AST) for standard-motive audio analytics.
The Huggingface dataset library gives a terrific interface for feeding custom statistics into these models for schooling purposes. further, there are extra than 70k public datasets to be had on the hugging Face hub. these consist of most popular datasets from the NLP (e.g. glue, imdb), CV (e.g. CIFAR, Mnist) and audio (e.g. speech instructions, audioset) area. Having a lot of these datasets available inside the standardized Hugging Face dataset layout makes it clean to pre-teach and to validate distinctive fashions.
Why know-how and curating your ML dataset is crucial
Hugging Face models may be tailored to custom issues either via first-rate-tuning or prompt engineering. For both applications it’s far key to very well understand the available records for the problem. In fact, experienced records scientists realize that expertise and enhancing the data (facts curation) is the most time consuming technique in an AI mission.
finding issues in unstructured records units (e.g. corner cases, fake labels) is typically a two-step process: First, an algorithmic heuristic is used to flag applicants and to pick out information clusters with bad overall performance. Then, these clusters and records factors are manually inspected. The latter part is state-of-the-art omitted. Greg Brockman (Founder and president modern OpenAI) writes:
“manual inspection trendy information has probable the highest cost-to-prestige ratio modern day any activity in machine state-of-the-art.” — Greg Brockman
what is Renumics spotlight ?
spotlight is a tool to interactively discover unstructured datasets from dataframes with just one line latest code. It helps you to create interactive visualizations, and leverage records enrichments to pinpoint crucial clusters for your information. we are constructing spotlight for groups that need to be today’s of their workflows and their tooling. spotlight gives:
help for maximum unstructured records types: images, audio, text, films, time-series, and geometric facts.
smooth integration: you could configure and start highlight with only a few lines ultra-modern code.
Customizable: you may create custom visualizations and inspection lenses in the GUI or with the Python API.
rich examples and use cases to leap-start your statistics exploration journey.
spotlight Hugging Face datasets
The Huggingface Datasets library has several functions that makes it an excellent tool for operating with ML datasets: It shops tabular data (e.g. metadata, labels) in conjunction with unstructured statistics (e.g. pictures, audio) in a not unusual Arrows table. Datasets also describes crucial facts semantics through features (e.g. pics, audio) and extra assignment-precise metadata.
spotlight at once works on top cutting-edge the datasets library. trendy there is no need to duplicate or pre-procedure the dataset for records visualization and inspection. highlight loads the tabular statistics into reminiscence to allow for efficient, consumer-facet records analytics. memory-in depth unstructured information samples (e.g. audio, snap shots, video) are loaded lazily on demand.
by using nature, a valid wave is a non-stop signal, meaning it incorporates an countless range modern day sign values in a given time. This poses issues for digital devices which assume finite arrays. To be processed, stored, and transmitted by means of virtual devices, the non-stop sound wave needs to be transformed into a series trendy discrete values, known as a digital illustration.
if you have a look at any audio dataset, you’ll discover virtual files with sound excerpts, which includes textual content narration or track. you can encounter specific report formats which includes .wav (Waveform Audio document), .flac (loose Lossless Audio Codec) and .mp3 (MPEG-1 Audio Layer 3). these formats in particular range in how they compress the virtual representation cutting-edge the audio signal.
allow’s take a look at how we arrive from a non-stop signal to this illustration. The analog sign is first captured by using a microphone, which converts the sound waves into an electrical sign. the electric signal is then digitized through an Analog-to-digital Converter to get the digital illustration via sampling.
Sampling and sampling rate
Sampling is the manner modern measuring the cost modern-day a continuous signal at constant time steps. The sampled waveform is discrete, since it incorporates a finite quantity ultra-modern sign values at uniform intervals.
Instance from Wikipedia article: Sampling (signal processing)
The sampling rate (additionally referred to as sampling frequency) is the range present day samples taken in one 2d and is measured in hertz (Hz). to provide you a point today’s reference, CD-nice audio has a sampling rate latest forty four,one hundred Hz, that means samples are taken 44,a hundred instances in keeping with 2d. For comparison, excessive-decision audio has a sampling fee present day 192,000 Hz or 192 kHz. A not unusual sampling charge used in education speech models is 16,000 Hz or sixteen kHz.
the choice present day sampling price in general determines the very best frequency that may be captured from the sign. that is additionally referred to as the Nyquist limit and is exactly 1/2 the sampling charge. The audible frequencies in human speech are below eight kHz and therefore sampling speech at sixteen kHz is enough. the usage of a better sampling fee will now not capture more records and simply results in an boom within the computational cost present day processing such documents. on the other hand, sampling audio at too low a sampling charge will bring about information loss. Speech sampled at eight kHz will sound muffled, as the higher frequencies cannot be captured at this price.
It’s important to ensure that each one audio examples on your dataset have the same sampling price when operating on any audio project. in case you plan to use custom audio information to satisfactory-song a pre-trained version, the sampling fee cutting-edge your statistics should match the sampling rate modern day the statistics the model was pre-skilled on. The sampling price determines the time c program languageperiod between successive audio samples, which affects the temporal resolution cutting-edge the audio data.
keep in mind an example: a 5-2d sound at a sampling charge ultra-modern 16,000 Hz will be represented as a chain ultra-modern 80,000 values, at the same time as the equal 5-2nd sound at a sampling fee state-of-the-art 8,000 Hz could be represented as a chain modern day 40,000 values. Transformer models that remedy audio tasks deal with examples as sequences and rely on attention mechanisms to analyze audio or multimodal representation. In view that sequences are different for audio examples at one of a kind sampling costs, it will be tough for fashions to generalize between sampling quotes. Resampling is the method of making the sampling charges fit, and is trendy preprocessing the audio data.
Amplitude and bit depth
at the same time as the sampling charge tells you how today’s the samples are taken, what precisely are the values in each pattern?
Sound is made with the aid of adjustments in air pressure at frequencies which are audible to people. The amplitude modern-day a legitimate describes the sound pressure degree at any given instantaneous and is measured in decibels (dB). We perceive the amplitude as loudness. to give you an instance, a normal speakme voice is below 60 dB, and a rock live performance can be at around one hundred twenty five dB, pushing the boundaries brand new human hearing.
In digital audio, every audio pattern information the amplitude ultra-modern the audio wave at a point in time. The bit intensity modern-day the pattern determines with how much precision this amplitude value can be described. The higher the bit depth, the extra faithfully the digital representation approximates the unique non-stop sound wave.
The most commonplace audio bit depths are 16-bit and 24-bit. each is a binary term, representing the variety cutting-edge viable steps to which the amplitude fee may be quantized when it’s transformed from non-stop to discrete: 65,536 steps for 16-bit audio, a whopping 16,777,216 steps for twenty-four-bit audio. because quantizing involves rounding latestf the non-stop cost to a discrete value, the sampling procedure introduces noise. The higher the bit depth, the smaller this quantization noise. In exercise, the quantization noise today’s 16-bit audio is already small enough to be inaudible, and the usage of better bit depths is typically now not vital.
you may additionally come upon 32-bit audio. This shops the samples as floating-factor values, whereas 16-bit and 24-bit audio use integer samples. The precision modern a 32-bit floating-point cost is 24 bits, giving it the same bit intensity as 24-bit audio. Floating-factor audio samples are expected to lie within the [-1.0, 1.0] range. considering that gadget learning fashions certainly paintings on floating-factor data, the audio must first be converted into floating-point format before it could be used to train the version. We’ll see the way to try this within the subsequent section on Preprocessing.
just as with continuous audio signals, the amplitude ultra-modern digital audio is normally expressed in decibels (dB). for the reason that human hearing is logarithmic in nature — our ears are more sensitive to small fluctuations in quiet sounds than in loud sounds — the loudness modern-day a sound is less difficult to interpret if the amplitudes are in decibels, that are additionally logarithmic. The decibel scale for real-international audio begins at zero dB, which represents the quietest possible sound human beings can hear, and louder sounds have large values. but, for digital audio alerts, zero dB is the loudest feasible amplitude, even as all other amplitudes are negative.
As a quick rule present day thumb: each -6 dB is a halving contemporary the amplitude, and anything underneath -60 dB is generally inaudible unless you genuinely crank up the extent.
Audio Data as a waveform
you can have seen sounds visualized as a waveform, which plots the pattern values through the years and illustrates the modifications inside the sound’s amplitude. this is additionally referred to as the time area representation trendy sound.
This today’s visualization is useful for figuring out particular capabilities modern-day the audio sign consisting of the timing contemporary character sound activities, the overall loudness latest the signal, and any irregularities or noise gift inside the audio.
to plot the waveform for an audio sign, we are able to use a Python library known as librosa:
Copied
pip installation librosa
allow’s take an example sound known as “trumpet” that includes the library:
Copied
import librosa
array, sampling_rate = librosa.load(librosa.ex(“trumpet”))
the instance is loaded as a tuple today’s audio time collection (right here we name it array), and sampling rate (sampling_rate). permit’s test this sound’s waveform with the aid of using librosa’s waveshow() characteristic:
Copied
import matplotlib.pyplot as plt
import librosa.show
plt.discern().set_figwidth(12)
librosa.display.waveshow(array, sr=sampling_rate)