Machine Learning on Sound and Audio data
Audio data
Audio Data. Most agencies have a gold mine of information: insights into operational activity, customer behavior, market performance, worker productivity, and much more. But having a variety of records is not always the same as having beneficial information and intelligence to assist in making vital decisions. Many corporations fail to use their data to its maximum capacity, a stark truth confirmed through our 2023 Innovation Index.
Using actionable insights and leveraging rapid delivery system intelligence and automation (specifically GenAI) across your employer relies on reliable and accessible records. Organizations that commit to driving decisions and automation using a single source of truth can be well positioned to perform well, innovate boldly, and seize a clean, aggressive lead.
The cost of being pushed to records:
Make short, confident decisions across the company.
Create operational efficiencies while reducing errors and charges.
Take advantage of the opportunities of independent, generative AI.
Build a statistical foundation to leverage the strength of factual information groups need to better navigate uncertainty, improve operational performance, and enable valuable experiences for customers, staff, and partners. However, before you can drive your organization’s value chain through the use of analytics, automation and artificial intelligence, you need to build a solid statistical foundation through:
- Accelerate business enterprise decision-making insights by ensuring statistics can be analyzed across all factors of the organization to enable record-centric decisions
- Distribute statistics across the organization by leveraging cutting-edge data governance technologies to drive Cost
- Leverage governance to enable trust in facts, ensuring that all corners of the corporation agree with available and available facts.
- Optimize movements with a man-machine association that balances human and temporal resources to create efficiencies in the company and reduce errors and costs in obtaining information. Excellence across culture and operations by prioritizing statistical resources so teams can recognize and leverage the value of that data to drive lifestyle sharing.
Simplify AI complexity to drive costs
Organizations want to jump straight into AI. But AI projects can be difficult given the market hype about GenAI and the speed of generational innovation. The stakes are high and the pressure is directly to launch and/or drive AI tasks that generate costs. The key is to simplify the complexity of AI by focusing on three critical success factors:
AI method.
24x7offshoring your AI strategy with your business strategy. If you’re talking to investors or shareholders about increasing profitability or white space business model opportunities, then that’s what your AI approach should focus on as well.
datasets for machine learning ai
Business use instances. Awareness of how to identify high-value business use cases and create expanded pathways to move from % to real global solutions that increase measurable cost.
Information is inspiration. Make data strategy the foundation of your AI initiative. Because without a consistent, localized database, you won’t be able to accomplish even the most expensive use cases.
What you will learn
How system knowledge allows you to experience extraordinarily high amounts of test statistics before delving into how ML can help during both phases of the test automation process.
Use system learning to make practical decisions palatable for releases.
With 24x7offshoring , teams or feature teams deliver new code and costs to clients almost every day.
How system learning can improve test balance over time
The only reason to invest time and resources in creating check automation is so that you can answer high-quality questions associated with commercial business threats.
Companies that implement continuous checkout within 24x7offshoring run a wide variety of checkout types more than once a day. With each verification run, the amount of verification data created grows significantly, making the selection method more difficult.
With artificial intelligence and systems learning, executives should be able to better analyze test data, recognize features and patterns, quantify business risks, and make decisions more quickly and consistently. Without the help of AI or machine data acquisition, work is error-prone, manual, and sometimes impossible.
Sound data
The big problem when starting with sound data is that, unlike tabular records or images, sound records are not easy to represent in a tabular layout.
As you may or may not know, images can easily be represented as arrays since they can be pixel-based. Each of the pixels has a value that indicates the intensity of black and white (or for color images you have a depth of crimson, green and blue one by one).
Sound, on the other hand, is a much more complex record format to work with:
Sound is a mixture of wave frequencies with specific intensities. It is very important to convert to some type of tabular or matrix statistics set before doing any system development.
Secondly, sound is a matter of time. It is actually more comparable to video data than images, as it has sound fragments from a certain period rather than a capture at a single moment in time.
What is audio evaluation?
Audio analysis is a process of reworking, exploring and interpreting audio signals recorded by digital devices. In order to obtain solid knowledge data, it applies a series of technologies, which consist of modern and deep algorithms. Audio testing has already gained wide adoption across numerous industries, from entertainment to healthcare to manufacturing. Below we will give you the most famous use cases.
Speech reputation
Speech popularity refers to the potential that current computer systems have to differentiate spoken words with natural language processing strategies. It allows us to manipulate computers, smartphones and other devices using voice commands and dictate text to machines instead of guiding input.
Voice popularity
Speech recognition is intended to perceive humans through the unique characteristics of their voices rather than isolating separate words. The approach finds programs in security systems for consumer authentication. For example, the 24x7offshoring biometric engine verifies employees and customers with the help of their voices in the banking area.
Music Reputation Tune Recognition is a popular feature in apps like 24x7offshoring that allows you to select unknown songs from a quick sample. Another new utility for music audio analysis is genre classification: say, Spotify runs its own set of rules to group tracks into categories (its database contains more than 5,000 genres).
Popularity of ambient sound.
Popularity of ambient sound. It specializes in the identification of current noises. They surround us, promising a group of modern benefits for the automotive and manufacturing industries. It is fundamental to the IoT program experience environment.
Systems like Audio Analytic ‘pay attention’ to events inside and outside your car, allowing the car to make changes as a way to increase driver safety. Another example is Bosch’s SoundSee technology, which can analyze system noise and facilitate predictive maintenance to monitor equipment health and avoid costly disasters.
Healthcare is another area where environmental reputation is useful. Provides non-invasive monitoring of the current distant patient to detect activities such as falls. In addition, analysis of coughs, sneezes, snoring and other ultra-modern sounds can facilitate early detection, find out a patient’s reputation, assess the degree of infection in public spaces, etc.
One such cutting-edge real-world evaluation use case is Sleep.ai, which detects teeth grinding and loud nocturnal breathing noises during sleep. The solution created by 24x7offshoring for a Dutch healthcare startup allows dentists to discover and diagnose bruxism to finally understand the reasons for this abnormality and treat it.
No matter what advanced sounds you look into, it all starts with an experience in new audio discs and their specific features.
What are audio logs?
Audio statistics represent analog sounds in digital form, preserving primary residences as new and original. As we know from school physics lessons, a valid is a wave of modern vibrations that travels through a medium such as air or water and finally reaches our ears. It has three key characteristics to consider when studying audio data: term, amplitude, and frequency.
The term is how long a positive sound lasts or, in other words, how many seconds it takes to complete a cycle of modern vibrations.
Amplitude is the depth of the sound measured in decibels (dB), which we understand as loudness.
The frequency measured in Hertz (Hz) suggests how many sound vibrations are produced according to 2d. Humans interpret frequency as a low or high pitch.
While frequency is an objective parameter, pitch is subjective. The human hearing range is between 20 and 20,000 Hz. Scientists say that today we perceive all sounds below 500 Hz as bass, such as the roar of an airplane engine. In turn, for us a high pitch is anything beyond 2000 Hz (for example, a whistle).
Audio data reporting formats are similar to text and images, audio is unstructured data, meaning it is not organized into tables with linked rows and columns. rather, it can store audio in various reporting codecs such as WAV or WAVE (waveform audio file format) developed through Microstrendyt and IBM.
It is a lossless or raw recording format, meaning it does not compress the original sound recording; AIFF (Audio Interchange Record Design) developed by Apple. Like WAV, it actually works with uncompressed audio; FLAC (Free Lossless Audio Codec) developed by Xiph.Org foundation that provides free multimedia codecs and software accessories. FLAC files are compressed without losing sound.
MP3 (mpeg-1 audio layer 3) developed by the Fraunhlaster Society in Germany and supported worldwide. It is the most common document layout because it makes it easier to track portable devices and send them back and forth over the network. Although mp3 compresses the audio, it offers acceptable and exceptional sound.
We recommend using aiff and wav files for evaluation, as they do not lose any information present in analog sounds. At the same time, keep in mind that none of these or any other audio files can be sent to latest generation devices at the same time. For audio to be understandable by computers, the information must go through a metamorphosis.
The basics of audio log transformation that you need to understand before delving into ultra-modern audio document processing, we want to introduce you to unique phrases that you can find at almost every step of our journey from sound data series to getting ML predictions . It’s worth noting that audio analysis involves working with images rather than listening.
A waveform is a basic visual representation, an audio signal that shows how an amplitude changes over years. The graph shows time on the horizontal (X) axis and amplitude on the vertical (Y) axis, but it doesn’t tell us what happens to the frequencies.
Audio data. In recent times, deep knowledge is increasingly used for the music design class: in particular, convolutional neural networks (CNN) that take as input a spectrogram considered as an image in which specific shapes are searched for.
Convolutional neural networks (CNN) are very similar to regular neural networks: they are made of neurons that have learnable weights and biases. each neuron receives some inputs, performs a dot product, and optionally follows it with a nonlinearity. The entire network still expresses a single differentiable scoring characteristic: from raw photo pixels on one side to elegance scores on the other. and they still have a loss feature (e.g. SVM/Softmax) in the final (fully related) layer and all the hints/hints we evolved to gain knowledge from regular neural networks are still observed.
So what changes? 24x7offshoring architectures specifically assume that entries are photographs, allowing us to code certain residences into the structure. This then makes the above function more environmentally friendly to implement and greatly reduces the variety of parameters within the network.
Parent Source
They are capable of detecting core features, which can then be combined with subsequent layers of the CNN architecture, resulting in the detection of novel applicable and complex higher-order capabilities.
The data set includes one thousand audio tracks every 30 seconds in length. It consists of 10 genres, each represented through 100 tracks. The tracks are all sixteen-bit 22050 Hz monophonic audio documents in .Wav format.
The dataset can be downloaded from the Marsyas website.
It consists of 10 genres, i.e.
- Blues
- Classic
- we of a
- Disco
- Hip hop
- Jazz
- metal
- Pop
- Reggae
- Rock
Each style incorporates one hundred songs. General data set: thousand songs.
Before moving forward, I would recommend using Google Colab to do everything related to neural networks because it is free and features GPU and TPU as execution environments.
Convolutional neural network implementation
Let us start building a CNN for the gender type.
First of all, load all the necessary libraries.
- import pandas as pd
import numpy as np - import numpy as np
from numpy import argmax - from numpy import argmax
import matplotlib.pyplot as plt - import matplotlib.pyplot as plt
%matplotlib online - %matplotlib
import booksa - import booksa online
import booksa.display - import booksa.display
import IPython.display - import IPython.display import import warnings
import random
Random OS import from PIL import photo import pathlib import csv #sklearn Preprocessing from sklearn .Model_selection import train_test_split
#Keras import keras import warnings warnings.filterwarnings(‘forget’) from keras import layers from keras.layers import Activation, Dense, Dropout, Conv2D, Flatten,
MaxPooling2D, GlobalMaxPooling2D, GlobalAveragePooling1D, AveragePooling2D, join, add from keras.models import Sequential from keras.optimizers import SGD
from keras.models import Sequential from keras.optimizers import SGD Now convert the audio statistics files into images in PNG format or essentially extract the spectrogram for each audio. We can use Librosa Python library to extract the spectrogram from each audio file.
Genres = ‘blues classic us club hiphop jazz metal pop reggae rock’.split()
for g in genres:
pathlib.direction(f’img_data/{g}’).mkdir(parents=proper, exist_ok=current)
for filename in os.listdir(f’./pressure/My power/genres/{g}’):
song name = f’./power/My force/genres/{g}/{filename}’
and, sr = booksa. load(song name, mono=real, period=five)
print(y.shape)
plt.specgram(y, NFFT=2048, Fs=2, Fc=zero, noverlap=128, cmap=cmap, sides=’ default’, mode=’default’, scale=’dB’);
plt.axis(‘off’);
plt.savefig(f’img_data/{g}/{filename[:-3].replace(“.”,””)}.png’)
plt.clf()
The code above will create an img_data directory containing all tagged snapshots within the style.
- discernpattern spectrograms of Disco, Classical, Blues and u. s. style respectively.
- Disco and Classic
- Blues and you. s.
Our next step is to split the data into the train set and check the set.
- installation break folders.
- pip install split-folders
- We can split the data into 80% on the training and 20% on the test set.
- import cut folders
# To more easily split it into an education and validation set, set a tuple to `ratio`, i.e. `(.eight, .2)`.
split-folders.ratio(‘./img_data/’, output=”./information”, seed=1337, ratio=(.eight, .2)) # defaults
The above code returns 2 directories to educate and verify the set within a given list.
Image magnification :
Image augmentation artificially creates training images through extraordinary processing methods or the combination of various processing, including random rotation, shifting, cutting and flipping, etc.
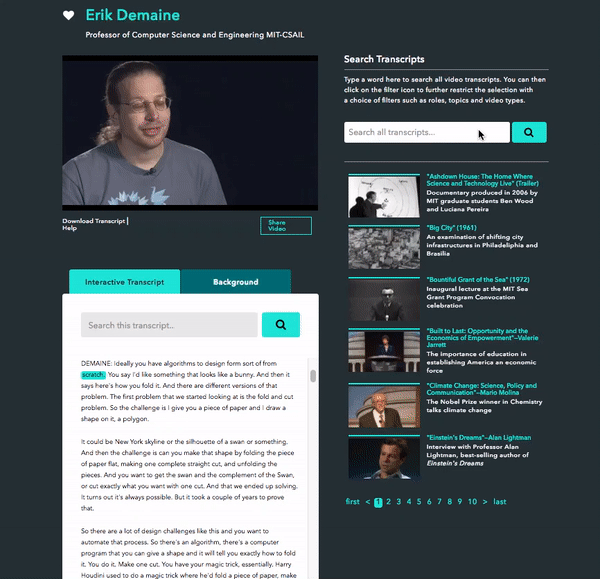
Perform image augmentation instead of educating your version with a large number of images. We can teach our model with fewer images and educate the version with exceptional angles and modify the images.
Keras has this elegant ImageDataGenerator that allows users to perform photo augmentation on the fly in an absolutely seamless manner. You can examine that during the authentic Keras documentation.
- de keras.preprocessing.photo importar ImageDataGenerator
train_datagen = ImageDataGenerator( - train_datagen = ImageDataGenerator(
rescale=1./255, # rescales all pixel values from zero to 255, so after this step all our pixel values are in the range (0,1) - rescale=1./255, # rescales all pixel values from zero to 255, so after this step all our pixel values are in the range (0,1)
shear_range=zero.2, # to use some random transformations - shear_range=zero.2, #to use some random transformations
zoom_range=0.2, #to zoom - zoom_range=0.2, #to zoom
horizontally_flip=true) # image can be flipper horiztest_datagen = ImageDataGenerator(rescale=1./255) - horizontal_flip=true) # image can be flipper horiztest_datagen = ImageDataGenerator(rescale=1./255)
ImageDataGenerator class has 3 strategies flow(), flow_from_directory() and flow_from_dataframe() to study images from a huge array and folders containing images.
we can only talk about flow_from_directory() in this blog post.
conjunto_entrenamiento = train_datagen.flow_from_directory(
‘./facts/educate’,
target_size=(64, sesenta y cuatro),
tamaño_por lotes=32,
class_mode=’express’,
shuffle = false)test_set = test_datagen.flow_from_directory(
‘./records/val’ ,
target_size=(sesenta y cuatro, 64),
lote_size=32,
class_mode=’categorical’,
shuffle = fake)
flow_from_directory() has the following arguments.
Listing: address where a folder exists, under which all test photos are located. For example, in this example, the training photos are determined in ./facts/teach
batch_size – set this to a small amount that accurately divides your total number of photos into your verification set.
Why is this simpler for test_generator?
Why is this easier for test_generator?
In fact, you should set the “batch size” on each teaching and valid turbine to a number that divides the total number of images for your teaching and valid set respectively, but this is not counted before because although the batch size does not It doesn’t accommodate the wide variety of samples in teaching or legitimate sets and some snapshots are overlooked every time we get the image from the generator, they will be sampled in the next epoch you train.
But for the test set, you should model the images exactly once, no less and no more. If you find this difficult, just set it to one (although maybe a little slower).
class_mode: Set to “binary” if you only have lessons to look forward to, if not set to “specific”, in case you are developing an Autoencoder tool, each input and output could be the same image, in this case. set to “input”.
shuffle: Set this to false, because you need to generate the snapshots in “order”, to predict the outputs and match them to their specific handles or file names.
Create a convolutional neural network :
- version = Sequential()
input_shape=(sixty-four, sixty-four, three)#1st hidden layer - input_shape=(sixty-four, sixty-four, three)#1st
model.add(Conv2D(32, (3, three), strides=(2, 2), input_shape=input_shape)) - modelo de capa oculta.add(Conv2D(32, (3, tres), strides=(2, 2), input_shape=input_shape))
version.add(AveragePooling2D((2, 2), strides=(2,2)) ) - version.add (AveragePooling2D((2, 2), strides=(2,2)))
model.add(Activation(‘relu’))#2d capa oculta - model.add(Activation(‘relu’))#2d
model.upload(Conv2D(sixty-four, (three, three), padding=”same”)) - hidden layer model.upload(Conv2D(sixty-four, (three, three), padding= “same”))
version.add(AveragePooling2D((2, 2), strides=(2,2))) - version.add(AveragePooling2D((2, 2), strides=(2,2)))
version.add(Activation(‘relu’))#3ra capa oculta - version.add(Activation(‘relu’))#3rd
model.upload(Conv2D(sixty-four, (3, 3), padding=”same”)) - hidden layer model.upload(Conv2D(sixty-four, (3, 3), padding=”same”))
model.add(AveragePooling2D((2, 2), strides=(2,2))) - model.add(AveragePooling2D((2, 2), strides=(2,2)))
model.add(Activation(‘relu’))#Flatten - model.add(Activación(‘relu’))#Flatten
model.upload(Flatten()) - model.upload (Flatten())
model.upload(Dropout(price=zero.5))#add absolutely connected layer. - model.upload(Dropout(price=zero.5))#add absolutely connected cover.
model.add(Dense(64)) - model.add(Dense(64))
version.upload(Activation(‘relu’)) - version.upload(Activation(‘relu’))
version.upload(Dropout(rate=0.five))#Output cover - version.upload(Drop(rate=0.five))#Capa de salida
version.add(Dense(10)) - version.add(Dense(10))
model.upload(Activation(‘softmax’))model.precis() - model.upload(Activation (‘softmax’))model.precis()
Gather/train the network using Stochastic Gradient Descent (SGD). Gradient Descent works exceptionally while we have a convex curve. However, if we do not have a convex curve, gradient descent fails. Therefore, in Stochastic Gradient Descent, few random samples are selected instead of the entire data set for each generation.
- epochs = two hundred
batch_size = 8 - batch_size = 8
learning_rate = zero.01 - learning_rate = zero.01
decay_rate = learning_rate / epochs - decay_rate = learning_rate /
momentum = 0.9 - epochs momentum = 0.9
sgd = SGD(lr=learning_rate, impulse=momentum, decay=decay_rate, nesterov=false) - sgd = SGD(lr=learning_rate, momentum=momentum, decay=decay_rate, nesterov=false)
model.compile(optimizer=”sgd”, loss=”categorical_crossentropy”, metrics=[‘accuracy’]) - model.compile(optimizer=”sgd”, loss=”categorical_crossentropy”, metrics=[‘accuracy’])
Now the model with 50 epochs is in shape.
- version.fit_generator(
training_set, - training_set,
steps_per_epoch = one hundred, - steps_per_epoch=one hundred,
epochs=50, - epochs = 50,
validation_data = test_set, - validation_data=test_set,
validation_steps=200) - validation_steps=200)
Now, since the CNN model is professional, let’s analyze it. evaluate_generator() uses both the input and output of its verification. It first predicts the result and usage of the training input and then evaluates the performance by comparing it with the test result. Therefore, it provides a measure of overall performance, i.e. accuracy where applicable.
#version evaluation model.evaluate_generator
(generator=test_set, steps=50)#OUTPUT
[1.704445120342617, 0.33798882681564246]
So the loss is 1.70 and the precision is 33.7%.
Otherwise, let your version make some predictions on the test data set. You want to reset test_set before each time you call predict_generator. This is critical, if you forget to reset test_set you may get results in an unusual order.
test_set.reset()
pred = model.predict_generator(test_set, pasos=50, detallado=1)
As of now predicted_class_indices has the anticipated labels, but you can’t really tell what the predictions are, because all you can see are numbers like 0,1,4,1,zero,6… You need to map the predictions labels with their precise IDs, including file names, so you know what it predicted for which photo.
- indexes_class_predichos=np.argmax(pred,eje=1)
- tags = (training_set.class_indices)
tags = dict((v,ok) for ok,v in tags.items()) - tags = dict((v,ok) for ok,v in tags.items())
predictions = [tags[k] for k in predicted_class_indices] - predictions = [tags[k] for k in predicted_class_indices]
predictions = predictions[:200] - predictions = predictions[:200]
filenames=test_set.filenames - filenames =test_set. file names
Add file names and predictions to a single pandas data frame as two separate columns. but before doing that check the dimensions of both, they must be the same.
print(len(filename, len(predictions)))
# (200, 2 hundred)
Then save the results to a CSV record.
results=pd.DataFrame({“Filename”:filenames,
“Predictions”:predictions},orient=’index’)
effects.to_csv(“prediction_results.csv”,index=false)
main outlet
I have trained the model in 50 epochs (which in turn took 1.5 hours to run on Nvidia K80 GPU). If you want to increase accuracy, increase the number of epochs to a thousand or more while training your version of CNN.
Therefore, it indicates that CNN is a viable opportunity for computerized feature extraction. This discovery helps our hypothesis that the intrinsic features in the variation of musical data are similar to those of photographic data. Our CNN model is exceptionally scalable but not strong enough to generalize the educational result to unseen music information. This will be achieved with an expanded data set and of course the amount of data set that can be fed.
Well, this concludes the series of two articles on audio statistics looking at using deep learning with Python. I hope you guys loved reading it, feel free to share your comments/minds/observations in the comments section.
We live in a world of trendy sounds: qualitative and worrying, low and excessive, quiet and loud, they affect our mood and our decisions. Our brains constantly process sounds to give us essential statistics about our environment. However, acoustic alerts can inform us even better if we analyze them with the use of cutting-edge technology.
Today, we have artificial intelligence and modern systems to extract information, inaudible to humans, from speech, voices, loud night breathing, noise from runways, businesses and visitors, and other modern acoustic alerts. In this article, we’ll share what we’ve discovered while creating fully robust AI-based reputation solutions for healthcare projects.
Specifically, we will explain how to obtain audio data, prepare it for analysis, and select the right ML model to achieve the highest prediction accuracy. But first, let’s go over the basics: what audio analysis is and what makes audio logs so difficult to approach.
What is audio analysis?
What is audio analysis?
Audio analysis is a process of reshaping, exploring and decoding audio indicators recorded using virtual devices. In order to obtain robust information statistics, it applies a series of technologies, including deep current algorithms. Audio analytics has already received wide adoption in various industries, from entertainment to healthcare to manufacturing. Below we will provide the most popular use cases.
speech reputation
speech reputation
The popularity of speech has to do with the ability of cutting-edge computers to differentiate spoken phrases with natural language processing techniques. It allows us to control PCs, smartphones and other devices using voice commands and dictate texts to machines instead of entering them manually. Siri through Apple, Alexa through Amazon, Google Assistant, and Cortana through Microslatett are famous examples of how deeply the generation has penetrated our daily lives.
voice reputation
voice reputation
Speech recognition is intended to identify humans with the help of specific features of their voices rather than isolating separate phrases. The method finds applications in protection structures for consumer authentication. For example, the Nuance Gatekeeper biometric engine verifies employees and customers using their voices in the banking area.
annotation services , image annotation services , annotation , 24x7offshoring , data annotation , annotation examples
musical popularity
Track popularity is a famous feature in contemporary apps like Shazam that allows you to discover unknown songs from a short pattern. Any other ultra-modern music audio evaluation software is category of style: say, Spotify runs its proprietary set of rules to group tracks into classes (its database contains more than five thousand genres).
Popularity of ambient sound
Ambient sound recognition specializes in identifying the latest noises around us, promising a host of current benefits for the automotive and manufacturing industries. It is crucial to understand the IoT packet environment.
Systems like Audio Analytic ‘listen’ to activities inside and outside your car, allowing the car to make modifications to increase driver safety. Another example is Bosch’s SoundSee technology that can analyze device noises and facilitate predictive maintenance to show device status and avoid costly disasters.
Healthcare is another topic where the popularity of ambient sound is achievable. It offers state-of-the-art, non-invasive remote patient monitoring to detect events such as falls. In addition to that, analyzing coughs, sneezes, snoring and other modern sounds can facilitate early detection, determine a patient’s reputation, assess infection status in public spaces, etc.
A real use case for this type of analysis is Sleep.ai, which detects teeth grinding and loud nighttime breathing noises at some point during sleep. The solution created with AltexScutting-Eget for a Dutch healthcare startup allows dentists to detect and monitor bruxism to then understand the causes of this abnormality and treat it.
Whatever modern sounds you analyze, it all starts with an understanding of modern audio data and its specific characteristics.
What are audio statistics?
What are audio statistics ?
Audio information represents analog sounds in virtual form, preserving the primary properties of ultra-modern and authentic. As we know in school physics classes, a real is a wave of modern vibrations that travels through a medium such as air or water and finally reaches our ears. It has three key characteristics to consider when studying audio records: time period, amplitude, and frequency.
Audio information represents analog sounds in virtual form, preserving the primary properties of ultra-modern and authentic. As we know in school physics classes, a real is a wave of modern vibrations that travels through a medium such as air or water and finally reaches our ears. It has three key characteristics to consider when studying audio records: time period, amplitude, and frequency.
The term is how long a given sound lasts or, in other words, how many seconds it takes to complete a cycle of contemporary vibrations.
Amplitude is the intensity of sound measured in decibels (dB) that we perceive as volume.
The frequency measured in Hertz (Hz) shows how many sound vibrations occur according to the 2nd. Humans interpret frequency as a low or high pitch.
While frequency is an objective parameter, pitch is subjective. The range of human listening is between 20 and 20,000 Hz. Scientists say that the latest advances consider all sounds below 500 Hz as serious, such as the roar of an airplane engine. In turn, for us the high tone is anything that exceeds 2000 Hz (for example, a whistle). Audio data reports codecs. Like text and images, audio is unstructured records, meaning it is not organized into tables with linked rows and columns. instead you can store audio in different file formats like
Instead, you can store audio in different file formats, such as WAV or advanced WAVE (Waveform Audio Report Design) through Microstrendyt and IBM. It is a lossless or raw report layout, meaning it does not compress the original sound recording; AIFF (Audio Interchange Reporting Format) developed by Apple. Like WAV, it works with uncompressed audio; FLAC (Free Lossless Audio Codec) evolved through the Xiph.Org foundation that offers free multimedia formats and software tools. FLAC files are compressed without losing excellent sound.
MP3 (mpeg-1 audio layer 3) developed by the Fraunhbrand Newer Society in Germany and supported worldwide. It is the most common disk design, as it makes the tune easy to store on portable devices and be sent back and forth over the network. Although mp3 compresses the audio, it still offers a pleasant and acceptable sound.
We recommend using aiff and wav files for analysis, as they do not lose any records found in analog sounds. At the same time, remember that none of these or other audio files can be immediately introduced into devices of modern models. For audio to be understandable to computers, the records must undergo a change.
Basic audio log transformation concepts you need to understand. Before we delve into state-of-the-art audio file processing, we need to introduce unique phrases that you’ll encounter at almost every step of our journey from robust statistical series to ML predictions. . It’s worth noting that audio analysis involves working with images rather than listening.
A waveform is a primary visual representation of an audio signal that shows how an amplitude changes over years. The graph shows time on the horizontal (X) axis and amplitude on the vertical (Y) axis, however it does not tell us what happens to the frequencies.
A modern example of a waveform. supply: Audio signal processing for modern machines
supply: Audio signal processing for modern machines
A spectrum or spectral graph is a graph in which the X-axis suggests the most recent frequency of the sound wave while the Y-axis represents its amplitude. This last visualization of sound data allows you to analyze the frequency content but omits the time component.
A new example of a spectrum graph. supply: Analytics Vidhya
Vidya Analytics
A spectrogram is an in-depth view of a signal that covers all three characteristics of the current sound. It can learn time from the x-axis, frequencies from the y-axis, and amplitude from color. The noisier the occasion, the brighter the color, while silence is represented in black. Having three dimensions on a graph can be very convenient: it allows you to hear how frequencies change over time, observe the sound in all its fullness, and detect different problem regions (like noises) and styles with the help of your eyes.
An example of an ultra-modern spectrogram. source: iZotope
A mel spectrogram in which mel means melody is a state-of-the-art spectrogram based on the mel scale that describes how humans understand sound traits. Our hearing can distinguish low frequencies better than high frequencies. You can try it yourself: try playing tones from 500 to 1000 Hz and then from 10,000 to 10,500 Hz. The first frequency range would seem much wider than the second, although in reality they are the same. The mel spectrogram includes this particular feature of ultra-modern human listening, changing the values in Hertz to the mel scale. This approach is widely used for genre classification, device detection in songs, and popularity of speech emotions.
The mel spectrogram includes this particular feature of ultra-modern human listening, changing the values in Hertz to the mel scale. This approach is widely used for genre classification, device detection in songs, and popularity of speech emotions.
A contemporary example of a mel spectrogram. supply: Devopedia
The Fourier transform (feet) is a mathematical function that decomposes a sign into peaks of different amplitudes and frequencies. We use it to convert waveforms into corresponding spectrum graphs to observe the same signal from a distinctive perspective and perform frequency evaluation. It is a powerful tool to understand indicators and fix errors in them.
Fast Fourier Reshaping (FFT) is the algorithm that calculates Fourier Reshaping.
Make use of FFT to view the equal signal from time and frequency perspectives. source: NTi Audio
The short-time Fourier transform (STFT) is a series of modern Fourier transforms that transform a waveform into a spectrogram.
Audio evaluation software
Of course, there is no need to perform transformations manually. You also don’t need to understand the complex mathematics behind ft, STFT, and other methods used in audio analysis. Many of these and many other tasks are routinely performed by audio analysis software that in most cases supports the following operations:
- import audio information
load annotations (tags), - upload annotations (tags),
edit recordings and split them into parts, - edit recordings and split them into pieces,
modern noise, - modern noise,
converting indicators into corresponding visible representations (waveforms, spectrum diagrams, spectrograms, fusion spectrograms),
Convert indicators into corresponding visible representations (waveforms, spectrum plots, spectrograms, fusion spectrograms), perform preprocessing operations, analyze time and frequency content, extract audio features, and more.
More advanced platforms also allow you to train modern device models and even provide you with pre-trained algorithms.
Here is the latest list of the most popular tools used in audio evaluation.
Audacity is a free and open source audio editor for splitting recordings, rendering noise, transforming waveforms into spectrograms, and labeling them. Audacity requires no coding talents. But its audio analysis toolset isn’t always cutting-edge. To take additional steps, you need to load your dataset into Python or transfer it to a specially specialized analysis platform and/or new device.
Tagging current audio data in Audacity. Offer: current information science.
Offer: current information science.
The Tensorflow-io package for practicing and improving modern audio data allows you to perform a wide variety of cutting-edge operations: noise removal, changing waveforms to spectrograms, frequency and time overlay to make sound safely audible and better. The tool belongs to the open source TensorFlow environment and covers the modern end-to-end device workflow. So after preprocessing you can train a version of ML on the same platform.
Librosa is an open source Python library that has almost everything you want for track and audio analysis. It allows you to display the characteristics of the latest audio files, expand all current audio information displays and extract functions from them, to name just a few.
Audio Toolbox through MathWorks offers numerous units for processing and analyzing audio data, from labeling to estimating sign metrics and extracting positive features. It also comes with pre-trained machine learning and entirely new models that can be used to analyze speech and sound popularity.
Audio data evaluation steps
Now that we have a basic understanding of modern sound information, let’s take a look at the contemporary key levels of the audio evaluation project from start to finish.