The role of a data annotator in machine learning
Data annotator
Data annotator. Information Annotation enrich your facts with our variety of human-annotation offerings at scale.
It’s surely now not sufficient to present a pc a huge quantity of statistics and count on it to research – facts has to undergo guidance for computers to discover styles and inferences within it. That’s in which we come in. We preprocess information to make it usable for device studying. “Annotation” refers to any metadata tag used to mark up factors of a dataset. adding significant metadata to the original dataset affords a layer of wealthy statistics to guide system gaining knowledge of.
Get entry to the information of qualified annotators with our community of 1 million+ AI professionals
We are able to speedy method hundreds of heaps of records rows so your fashions get the facts they need to work inside the real world. We harness the intelligence, capabilities, and cultural information from our international community of individuals to create the best nice information.
Within the technology of artificial intelligence and device gaining knowledge of, information annotation has emerged as a crucial method.
This newsletter delves into the position of a records annotator, an regularly-underestimated professional who aids in training AI systems by labeling and categorizing facts.
We discover the skills required, the importance of this role in the AI area, its practical applications, and discuss capability demanding situations and answers inside the field of records annotation.
knowledge the function of a records Annotator
The essence of a facts annotator’s position lies inside the meticulous processing and labeling of information, which serves because the bedrock for developing and refining system learning fashions. As a critical player in the statistics pipeline, a records annotator is entrusted with the undertaking of creating annotations that offer context and that means to uncooked facts.
The annotation method is an difficult one, requiring precision and attention to element. statistics annotators are expected to produce 86f68e4d402306ad3cd330d005134dac annotated records that can be used to educate machine learning algorithms. The accuracy of annotation is paramount, as any inaccuracies can compromise the validity of the device getting to know model.
Annotation analysts paintings closely with statistics annotators, overseeing the annotation techniques used and making sure that the very best standards are maintained. They scrutinize the excellent of the annotations, making sure that they’re comprehensive, applicable, and correct.
The technique of records Annotation explained information annotation, a complex and multifaceted technique, involves the software of labels to uncooked facts and, on the identical time, requires a deep know-how of the situation count to ensure accuracy and relevance.
The process of information annotation explained here revolves in general around using annotation software program, which assists annotators in labeling data based totally on pre-established annotation pointers. those tips provide a framework for the way the annotation count number have to be treated to hold consistency across the board. Annotators, then, use this framework to use labels to the statistics, remodeling it from an unstructured mass into an prepared set of records.
This labeling of records is crucial inside the development of machine learning fashions and synthetic intelligence algorithms, which depend on annotated records to learn and expect future outcomes.
Human-handled records annotation is regularly favored over automated methods. that is due to the fact human facts annotators own the capability to recognize context, nuances, and complicated instances higher, main to more accurate and applicable annotations.
The complete procedure, therefore, even as complex and disturbing, plays a crucial function in driving the advancement of technology.
Capabilities Required to turn out to be a facts Annotator acquiring proficiency as a records annotator needs a mix of technical knowledge and gentle abilties, each of which make contributions to the meticulous and nuanced mission of statistics annotation.
To correctly aid machines in sample recognition and know-how, a facts annotator must have a deep know-how of semantic annotation. This entails marking records with metadata that aids in purpose annotation, hence assisting machines apprehend the context and which means behind facts.
- To turn out to be a talented records annotator, the subsequent abilties are critical:
- A sturdy knowledge of language models: This allows annotators to interpret and annotate records as it should be, assisting machines recognize textual content, speech, or different data forms.
- Skillability in semantic segmentation: This talent includes dividing facts into segments, each carrying a selected that means.
- Familiarity with a crowdsourcing platform: this is essential as many records annotation obligations are done on these systems.
- Sturdy interest to detail: this is pivotal to make sure , errors-unfastened annotations.
The importance of records Annotation in AI and machine studying within the realm of synthetic intelligence and gadget learning, both of which closely rely upon information, the function of unique and complete statistics annotation can’t be overstated. information annotation serves as the cornerstone of those disciplines, forming the inspiration upon which superior algorithms and predictive models are built.
The importance of records annotation is exceptional validated while thinking about its software in various sectors. as an instance, in the improvement of self-driving motors, records annotation groups meticulously label and categorize limitless photos and sensor readings, teaching the AI how to interpret and respond to one-of-a-kind situations on the street.
Further, inside the realm of finance, data annotation is fundamental to knowledge complicated marketplace developments and patterns. here, finance information annotation is utilized to create advanced models able to predicting stock marketplace moves and monetary developments.
In social media analytics, sentiment annotation is employed to recognize human emotions and online behaviors, allowing corporations to tailor their strategies for that reason. The equal degree of precision is required in commercial facts annotation, in which properly annotated records can extensively enhance performance and productiveness in manufacturing approaches.
Everyday applications of information Annotation whilst many won’t realise it, truly every factor of our digital lives is prompted by the paintings of statistics annotators. those at the back of-the-scenes experts play a essential function in shaping the digital surroundings round us.
The paintings of facts annotators is broadly carried out in numerous ordinary programs. right here are a few examples:
Social Media: information annotation is used to create algorithms for customized content material idea, permitting systems like facebook and Instagram to propose posts and advertisements based totally in your possibilities.
Online buying: It helps in product advice structures, making your online purchasing enjoy more personalised by suggesting items that align along with your beyond purchases.
Healthcare: inside the healthcare zone, annotated facts assists in diagnosing sicknesses from scientific pics, enhancing affected person care.
Autonomous motors: information annotators help train self reliant riding structures to recognize and respond to one-of-a-kind avenue symptoms, pedestrians, and other motors, improving protection on the roads.
Through those programs, and many greater, statistics annotation notably impacts our digital stories. It shapes how we interact with technology on a every day foundation, and continues to accomplish that as technology evolves.
A deeper expertise of this technique helps us respect the regularly-unnoticed paintings of data annotators.
Capability challenges and solutions in statistics Annotation records annotation, no matter its critical position in shaping our virtual global, offers a completely unique set of demanding situations, and information these limitations is key to developing effective solutions.
One of the primary hurdles is retaining the accuracy and consistency of annotations, which can be compromised via human errors or differing interpretations amongst information annotators. A capacity answer is the implementation of strict hints and normal fine exams to make sure high standardization.
Some other mission is facts privateness, especially when coping with sensitive statistics. Annotators frequently need get entry to to personal facts, that can lead to privateness breaches if no longer dealt with efficaciously. One solution is to anonymize information before it’s far annotated, thereby shielding person identities.
Moreover, scalability may be a difficulty as gadget getting to know fashions frequently require sizable quantities of annotated records. guide annotation may be time-ingesting and luxurious. To fight this, agencies can rent computerized annotation tools. however, these tools are not best, so a human-in-the-loop technique is frequently preferred.
Lastly, language and cultural nuances also can pose a challenge in facts annotation. this is especially apparent in natural Language Processing initiatives. A capacity answer is to interact local audio system or cultural professionals within the annotation manner. Doing so can help to mitigate misinterpretations and biases.
Bringing the destiny closer to Us
The function of a information annotator is becoming more and more reported inside the realm of synthetic intelligence and gadget gaining knowledge of. Their job of adding metadata to statistics sets requires precision and analytical abilties, and has large packages in our virtual technology.
consequently, the significance of records annotation and annotators will continue to grow as we advance in era.
What is information annotation and why is information crucial?
Subscribe the two synonymous terms “statistics annotator” and “records labeler” seem to be anywhere in recent times. however who is a statistics annotator? Many know that annotators are somehow related to the fields of artificial Intelligence (AI) and machine mastering (ML), and that they likely have important roles to play in the information labelling market. however now not each person fully is aware what facts labelers honestly do.
What’s facts annotation and why is facts essential?
Information annotation is the process of labeling factors of statistics (snap shots, films, textual content, or some other format) by including contextual records which ML models can learn from. It helps ML fashions recognize what exactly is vital about every piece of records.
To completely hold close and recognize the entirety information labelers do and what information annotation talents they want, we need initially the fundamentals by means of explaining information annotation and statistics usage within the area of gadget learning. So, allow’s start with something extensive to offer us appropriate context after which dive into greater narrow strategies and definitions.
Statistics comes in lots of unique paperwork – from snap shots and motion pictures to textual content and audio documents – but in nearly all cases, this statistics must be processed to render itself usable. What it means is that this information has to be organized and made “clear” to whomever is the use of it, or as we are saying, it has to be “labeled”.
If, as an instance, we’ve got a dataset complete of geometric shapes (data factors), to prepare this dataset for in addition use, we need to ensure that every circle is categorized as “circle,” each rectangular as “square,” each triangle as “triangle,” and so on.
This turns a random series of gadgets in the dataset into something with a system that may be picked up and inserted right into a real-life task, a bunch of training statistics for a device getting to know algorithm. the alternative of it’s miles “raw” facts, which is basically a mass of disorganized facts. And this is in which the information annotator role is available in: those human beings turn “uncooked information” into “categorised facts”.
Facts annotation in machine gaining knowledge of models
This processing and business enterprise of uncooked unstructured records – “statistics labeling” or “facts annotation” – is even greater important in enterprise. when your commercial enterprise is based on facts in any way (that’s becoming increasingly not unusual today), you simply can’t manage to pay for for your facts to be messy, otherwise your enterprise will in all likelihood run into extreme troubles or fail altogether.
Categorised facts can help many distinct groups, both large and small, whether those organizations rely upon ML technologies, or don’t have anything to do with AI. as an example, a real-property developer or a motel govt may also need to make a selection selection approximately constructing a brand new facility.
But earlier than making an investment, they want to perform an in-intensity analysis with a view to recognize what styles of lodging get booked, how fast, at some point of which months, and so on. All of that implies particularly prepared and “categorised” statistics (whether it’s referred to as that or now not) that may be visualized and utilized in selection-making.
A training algorithm (also known as system gaining knowledge of algorithm or ML version) is essentially smart code written by software program engineers that tells an AI answer the way to use the information it encounters. The technique of education device learning fashions entails numerous ranges that we won’t cross into right now.
But the major factor is this: each and each system getting to know version requires appropriately categorized information at multiple points in its existence cycle. And normally now not just some training records – lots of it! Such ground reality facts is used to train an ML model to start with, in addition to to reveal that it continues to produce accurate consequences over time.
AI-based programs: why can we need a machine getting to know version?
These days, AI products are no longer the stuff of fiction or even some thing niche and specific. most of the people use AI products on a ordinary foundation, perhaps with out even figuring out that they’re handling an ML-subsidized solution. probable one of the great examples is when we use Google Translate or a comparable internet carrier.
Assume ML fashions, suppose records annotations, suppose education and test statistics. Feel like asking Siri or Alexa some thing? It’s the equal deal again with virtual assistants: training algorithms, classified information. using someplace and having an internet map service lay out and narrate a course for you? yes, you guessed it!
A few other examples of disrupting AI technologies encompass self-driving motors, on-line purchasing and product cataloging (e-commerce), cyber protection, moderating critiques on social media, economic buying and selling, prison assistance, interpretation of scientific effects, nautical and area navigation, gaming, and even programming among many others.
No matter what enterprise an AI answer is made for or what area it falls below (as an instance, laptop imaginative and prescient that deals with visual imagery or natural Language Processing/NLP that offers with speech) – they all mean continuous information annotation at nearly every flip. And, of route, that means having people handy who can carry out human powered information annotation.
Statistics annotation techniques and brands records annotation may be performed in a number of approaches by means of utilizing specific “strategies”:
Statistics may be labeled through human annotators.
It is able to be classified synthetically (the use of machine intelligence).
Or it can be categorised in a “hybrid” manner (having each human and device functions).
As of proper now, human-handled facts annotation remains the most sought-after approach, because it has a tendency to supply the best nice datasets. ML tactics that involve human-dealt with information annotation are often known as being or having “human-in-the-loop pipelines.”
In relation to the facts annotation method, methodologies of acquiring manually annotated schooling facts range. one in all them is to label the data “internally,” this is, to apply an “in-residence” team. in this situation, as typical, the organization has to write down code and build an ML model on the center in their AI product. however then it also has to prepare training datasets for this machine learning model, regularly from scratch. even as there are benefits to this setup (particularly having complete control over each step), the principle drawback is that this tune is usually extremely costly and time-consuming.
The motive is that you have to do the whole thing your self, together with training your group of workers, finding the right information annotation software program, gaining knowledge of high-quality manage techniques, and so forth.
The alternative is to have your information labeled “externally,” that’s referred to as “outsourcing.” Creators of AI merchandise may additionally outsource to individuals or complete corporations to carry out their records annotation for them, which may contain one-of-a-kind stages of supervision and mission control. In this situation, the tasks of annotating facts are tackled by specialised businesses of human annotators with relevant revel in who often work within their chosen paradigm (for instance, transcribing speech or running with image annotation).
In a manner, outsourcing is a bit like having your personal outside in-residence group that you rent briefly, except that this crew already comes with its very own set of information annotation tools. whilst attractive to a few, this technique can also be very pricey for AI product makers. What’s more, information pleasant can regularly fluctuate wildly from mission to undertaking and crew to team; in spite of everything, the entire statistics annotation method is handled with the aid of a third party. And whilst you spend a lot, you need to make sure you’re getting your cash’s well worth.
Crowdsourced facts annotation
There’s additionally a type of massive-scale outsourcing referred to as “crowdsourcing” or “crowd-assisted labeling,” that is what we do at Toloka. The logic here is easy: in preference to relying on constant groups of statistics labelers with constant skill units (who’re regularly primarily based in one location), alternatively, crowdsourcing relies on a huge and numerous network of records annotators from all around the globe.
In assessment to different facts labeling methodologies, annotators from the “worldwide crowd” pick what precisely they’re going to do and whilst precisely they desire to contribute. some other big difference between crowdsourcing and all different processes, each internal and outside, is that “crowd contributors” (or “Tolokers” as we name them) do not need to be specialists or even have any experience in any respect. this is viable because:
A brief, undertaking-oriented schooling path takes place before each venture in labeling information – best folks that carry out check obligations at a excellent stage are allowed to continue to actual mission obligations.
Labeling companies 24x7offshoring
Crowdsourcing utilizes superior “aggregation techniques,” which means that it’s now not so much about character efforts of crowd individuals, however as a substitute approximately the “gathered attempt” of all and sundry on the records annotation assignment.
To apprehend this higher, consider it as painting a giant canvas. while in-house or outsourced teams step by step paint a whole image, relying on their knowledge and tenacity, crowd members as an alternative paint a tiny brush stroke every. In reality, the equal brush stroke in terms of its function on the canvas is painted via numerous members. this is the reason why an person mistake isn’t damaging to the very last end result. A “statistics annotation analyst” (a special type of ML engineer) then does the following:
They take every contributor’s enter and discard any “noisy” (i.e., low-pleasant) responses.
They mixture the effects by way of placing all of the overlapping brush strokes collectively (to get the first-class model of every brush stroke).
They then merge distinct brush strokes together to receive a complete picture. Voila – right here’s our geared up canvas!
Being a crowd contributor: what is information annotator activity?
This technique serves people who need annotated statistics very well, but it also makes records annotation loads less tedious for human annotators. probably the best aspect about being a data annotator for a crowdsourcing platform like Toloka is that you could paintings any time you want, from any location you preference – it’s absolutely as much as you. you could also paintings in any language, so talking your native tongue is more than enough. In case you communicate English collectively with some other language (native or non-local), that’s even higher – you’ll have the ability to participate in greater labeling tasks.
Every other incredible issue is that all you need is net access and a tool such as a cellphone, a tablet, or a computer/laptop pc. nothing else is needed, and no prior revel in is wanted, due to the fact, as we have explained already, task-specific education is furnished earlier than each labeling project.
Really, if you have know-how in a few field, this could handiest assist you, and you could also be asked to evaluate other participants’ submissions primarily based on your performance. What you produce can also be dealt with as a “golden” set (or “honeypot” as we say at Toloka), that is a popular that the others might be judged against.
All annotation responsibilities at Toloka are notably small, because ML engineers decompose massive labeling initiatives into greater workable segments. As a end result, irrespective of how tough the real request to label records made by our customer, as a crowd contributor, you’ll most effective ever need to address micro duties.
The primary issue is following your commands to the word. you need to be cautious and diligent when you label the facts. The duties are commonly pretty clean, but to do them well, one desires to remain targeted at some stage in the entire labeling technique and keep away from distractions.
Forms of facts annotation tasks there are many exceptional labeling responsibilities for crowd participants to select from, but they all fall into these two categories:
on-line responsibilities (you complete the whole lot on your device without visiting everywhere in man or woman)
Offline tasks, also known as “discipline” or “feet-on-road” obligations (you tour to goal places to finish labeling assignments).
While you choose to take part in a discipline task, you’re requested to visit a particular area to your location (commonly your city or your community) to complete a brief on-website online venture.
This mission ought to involve taking pics of all bus stops in the place, monuments, or espresso shops. it can also be something more elaborate like following a selected route within a shopping center to decide how lengthy it takes or counting and marking benches in a park. The outcomes of those responsibilities are used to improve web mapping offerings, in addition to brick-and-mortar retail (i.e., physical stores).
Online assignments have an expansion of programs, some of which we stated earlier, and they will encompass text, audio, video, or image annotation. every ML application incorporates several common project codecs that our customers (or “requesters” as we say at Toloka) regularly ask for.
Text annotation
Text annotation duties usually require annotators to extract precise records from herbal language statistics. Such classified statistics is used for training NLP (natural language processing) models. NLP models are used in search engines, voice assistants, automated translators, parsing of textual content documents, and so forth.
Text type
In such responsibilities (additionally known as text categorization) you may want to answer whether the textual content you notice suits the topic provided. for instance, to see if a seek query fits search engine outcomes — such data facilitates improve search relevance. it can also be a easy sure/no questionnaire, or you could want to assign the text a specific category. for instance, to decide whether the text consists of a query or a purchase purpose (this is also referred to as rationale annotation).
What are facts Annotators?
Facts annotators are people liable for labelling and tagging information used to train device learning models. They meticulously evaluation and interpret information, adding annotations, labels, and metadata that assist AI algorithms apprehend styles and make correct predictions. facts annotation includes diverse duties, including image and video class, item detection, sentiment analysis, speech recognition, and natural language processing.
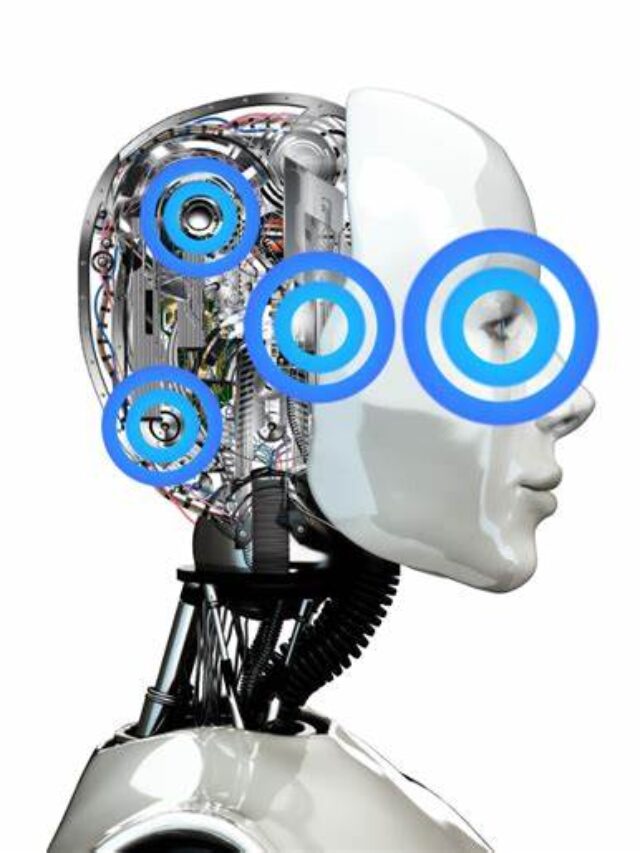
The significance of statistics Annotation:
Information annotation is a labour-intensive and crucial method in gadget getting to know. The best and accuracy of annotated statistics at once influence the performance and reliability of AI models. facts annotators play a pivotal position in growing education datasets that allow ML algorithms to examine and make correct predictions. right here are a few key motives why information annotation is critical:
Education ML fashions: gadget mastering models require big quantities of labelled facts to learn styles and make predictions. Annotators create training datasets by using meticulously labelling and annotating records, permitting ML algorithms to learn from various examples. these datasets are the foundation upon which AI structures are built.
Improving version performance: The accuracy of annotations directly impacts the overall performance of ML fashions. Records annotators make sure that annotations are specific, constant, and representative of actual-global situations. properly-annotated data lets in ML models to generalise styles efficaciously, main to advanced overall performance and robustness.
Handling complex information types: AI systems operate on diverse forms of facts, together with snap shots, text, audio, and video. Annotators own domain understanding and are skilled at decoding and labelling complicated records kinds as it should be. They understand the nuances and context associated with the statistics, permitting ML fashions to comprehend the subtleties vital for accurate predictions.
Mitigating Bias: Bias in AI algorithms is a giant difficulty. Annotators play a important position in mitigating bias by means of making sure that the labelled information is diverse, inclusive, and representative of various demographics and perspectives. They follow hints and protocols to minimise bias and provide a balanced and fair representation of the records.
The paintings of information annotators can be disturbing and time-consuming. A few not unusual demanding situations faced via annotators encompass:
Subjectivity and Ambiguity: decoding records and adding annotations can contain subjective judgment calls, especially in duties like sentiment evaluation or picture type. information annotators ought to adhere to suggestions and keep consistency while navigating ambiguous conditions.
Understanding and education: statistics annotators require area expertise and education to correctly label and annotate facts. They want to recognize the context and nuances related to the facts they may be annotating. non-stop gaining knowledge of and ability development are important for annotators to stay abreast of evolving AI technology and annotation strategies.
Time Constraints: constructing annotated datasets is a time-consuming technique. statistics annotators often face tight deadlines even as making sure accuracy and exceptional. Balancing velocity and precision can be tough, and it calls for powerful time management abilities.
Information privacy and protection: facts annotators take care of sensitive and personal information at some point of the annotation procedure. making sure facts privateness and protection is paramount, and annotators ought to adhere to strict protocols and hints to defend the confidentiality of the information they work with. They follow strict protocols to maintain records privacy and safety, making sure that the facts they take care of is protected from unauthorised get entry to or breaches.
The impact of facts Annotators on AI development
The contributions of statistics annotators to AI development can’t be overstated. Their meticulous paintings lays the inspiration for building strong and correct AI structures. here are a few ways in which annotators have a tremendous impact:
Enabling training and iteration: statistics annotators provide the labelled datasets which are critical for education ML models. without their efforts, AI algorithms could lack the important records to learn patterns and make predictions. Annotators additionally play a important function within the iterative procedure of AI improvement. They evaluate and refine annotations based on feedback from model overall performance, constantly improving the accuracy and reliability of ML algorithms.
Enhancing AI overall performance and Generalisation: annotations created by means of information annotators make a contribution to progressed AI overall performance. correct annotations enable ML fashions to generalise styles successfully and make correct predictions on unseen facts. Annotators make sure that the training datasets cover diverse eventualities and seize edge cases, allowing AI systems to perform nicely in real-world situations.
First-class control and Validation: records annotators are answerable for keeping the best and integrity of annotated datasets. They carry out rigorous great manipulate checks, verifying the accuracy and consistency of annotations. by means of ensuring that annotations align with mounted hints and requirements, annotators assist minimise mistakes and enhance the reliability of AI fashions.
Bias Mitigation and fairness: Addressing bias in AI algorithms is vital for moral and fair AI improvement. records annotators play a pivotal position in mitigating bias through carefully thinking about factors such as illustration, inclusivity, and equity for the duration of the annotation technique. by using supplying numerous and balanced annotations, annotators contribute to the improvement of AI systems that are extra equitable and independent.
Area expertise and Contextual understanding: statistics annotators bring treasured area knowledge to the annotation manner. Their know-how of the problem be counted facilitates in appropriately interpreting and labelling statistics. whether or not it’s medical photographs, criminal documents, or monetary records, annotators possess the vital know-how to annotate information successfully, permitting ML models to make knowledgeable selections in precise domain names.
non-stop gaining knowledge of and improvement: facts annotators are continuously getting to know and evolving along side improvements in AI technology. They live updated with the brand new annotation strategies, gear, and suggestions. This non-stop learning allows annotators to conform to changing requirements and enhance their annotation capabilities, ultimately enhancing the exceptional and relevance of annotated datasets.