WHAT IS DATA ANNOTATION
What is Data Annotator? what is data annotation
Building an AI or ML model that acts like a human requires enormous volumes of preparing information. For a model to settle on choices and make a moveannotator it should be prepared to comprehend explicit annotation annotation services , image annotation services , annotation , 24x7offshoring.
Information explanation is the order and marking of information for AI applications annotator.
Preparing information should be appropriately classified and clarified for a particular use case. With top caliber, human-fueled information comment, organizations can fabricate and improve AI executions data annotation annotation services , image annotation services , annotation , 24x7offshoring.
The outcome is an improved client experience arrangement like item suggestions, important web index results, PC vision, discourse acknowledgment, chatbots, and then some.
We have study what is data annotation , now There are a few essential sorts of information: text, sound, picture, and video
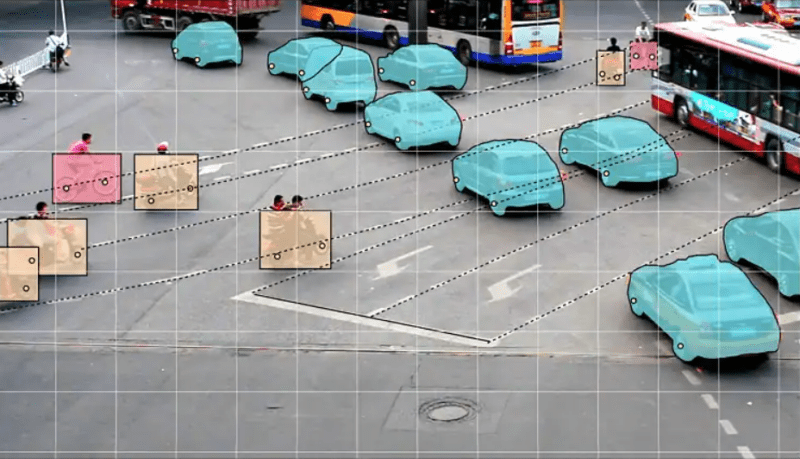
Text data Annotation
The most normally utilized information type is text – as indicated by the 2020 State of AI and Machine Learning report, 70% of organizations depend on text. Text explanations incorporate a wide scope of comments like feeling, goal, and question.
Supposition data Annotation
Supposition examination evaluates mentalities, feelings, and conclusions, making it essential to have the correct preparing information data annotation annotation services , image annotation services , annotation , 24x7offshoring.
To get that information, human annotators are frequently utilized as they can assess conclusion and moderate substance on all web stages, including web-based media and e-commerce locales, with the capacity to tag and give an account of watchwords that are indecent, touchy, or eulogistic, for instance.
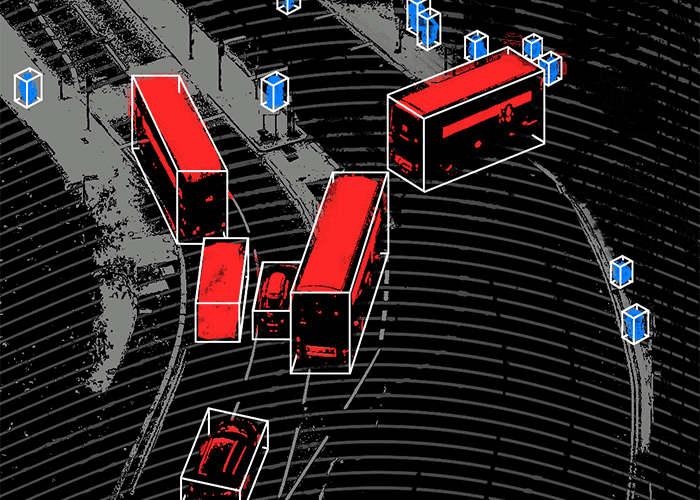
Plan Annotation
As individuals chat more with human-machine interfaces, machines should have the option to comprehend both regular language and client plan. Multi-expectation information assortment and classification can separate plan into key classes including demand, order, booking, suggestion, and affirmation.
Semantic comment both improves item postings and guarantees clients can discover the items they’re searching for. data annotator
These aides transform programs into purchasers. By labeling the different segments inside item titles and search questions, semantic explanation administrations help train your calculation to perceive those individual parts and improve in general inquiry significance.
Named Entity Annotation
Named Entity Recognition (NER) frameworks require a lot of physically commented on preparing information.
Associations like 24x7offshoring.com apply named substance comment capacities across a wide scope of utilization cases, for example, helping e-commerce customers recognize and label a scope of key descriptors, or supporting online media organizations in labeling elements like individuals, places, organizations, associations, and titles to help with better-focused on promoting content.
Sound Annotation
Sound explanation is the record and time-stepping of discourse information, including the record of explicit articulation and inflection, alongside the recognizable proof of language, tongue, and speaker socioeconomic.
Each utilization case is unique, and some require an unmistakable methodology:
For instance, the labeling of forceful discourse markers and non-discourse seems as though glass breaking for use in security and crisis hotline innovation applications data annotator .
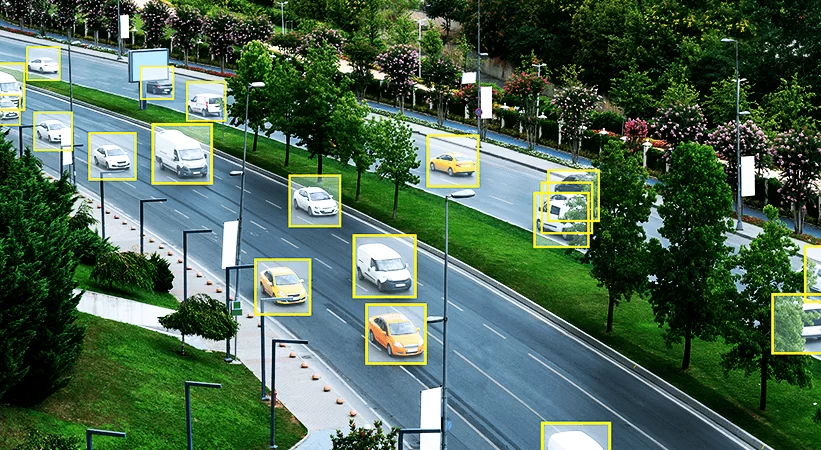
Genuine Use Case:
- Dial pad’s record models influence our foundation for sound record and classification
- Dial pad improves discussions with information data annotator.
They gather telephonic sound, translate those discoursed with in-house discourse acknowledgment models, and utilize characteristic language preparing calculations to fathom each discussion.
Picture Annotation
Picture comment is crucial for a wide scope of utilization, including PC vision, mechanical vision, facial acknowledgment, and arrangements that depend on AI to decipher pictures.
To prepare these arrangements, metadata should be appointed to the pictures as identifiers, subtitles, or catchphrases.
Constructing an AI or ML model that acts like a human requires big volumes of training data. For a design to make decisions and do something about it, it should be trained to understand particular info. Information annotation is the categorization and labeling of information for AI applications. Training information should be effectively classified and annotated for a specific use case. With high-quality, human-powered information annotation, companies can build and improve AI applications. The result is an enhanced client experience option such as item recommendations, pertinent search engine results, computer vision, speech recognition, chatbots, and more. There are numerous main kinds of data: text, audio, image, and video data annotator
Text Annotation
The most typically used data type is text– according to the 2020 State of AI and Machine Learning report, 70% of business rely on text. Text annotations include a vast array of annotations like sentiment, intent, and query.
Sentiment Annotation
Belief analysis examines mindsets, feelings, and viewpoints, making it important to have the best training data. To get that information, human annotators are often leveraged as they can assess belief and moderate content on all web platforms, consisting of social networks and eCommerce websites, with the ability to tag and report on keywords that are profane, sensitive, or neologistic, for example.
Intent Annotation
As individuals speak more with human-machine interfaces, devices should be able to comprehend both natural language and user intent. Multi-intent information collection and categorization can separate intent into crucial classifications including request, command, reservation, recommendation, and confirmation.
Semantic Annotation
Semantic annotation both improves item listings and ensures customers can discover the products they’re trying to find. This helps turn browsers into buyers. By tagging the different components within product titles and search queries, semantic annotation services help train your algorithm to recognize those specific parts and enhance total search importance.
Called Entity Annotation
Named Entity Recognition (NER) systems require a big quantity of manually annotated training information. Organizations like Appen use called entity annotation abilities throughout a vast array of use cases, such as helping eCommerce clients determine and tag a variety of key descriptors, or assisting social media companies in tagging entities such as people, places, business, organizations, and titles to assist with better-targeted marketing content.
Real Life Use Case: Improving Search Quality for Microsoft Bing in Multiple Markets
Microsoft’s Bing search engine required large-scale datasets to continuously enhance the quality of its search engine result– and the results needed to be culturally pertinent for the international markets they served. We delivered outcomes that surpassed expectations. Beyond delivering project and program management, we supplied the capability to proliferate in new markets with premium data sets. (Read the complete case study here).
Audio Annotation.
Audio annotation is the transcription and time-stamping of speech data, consisting of the transcription of particular pronunciation and intonation, in addition to the identification of language, dialect, and speaker demographics. Every usage case is various, and some need a really particular approach: for example, the tagging of aggressive speech indicators and non-speech seem like glass breaking for use in security and emergency situation hotline technology applications.
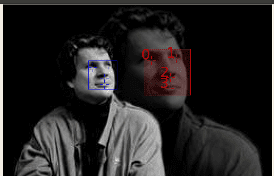
Real Life Use Case: Dialpad’s transcription designs utilize our platform for audio transcription and classification.
Dialpad enhances conversations with information. They gather telephonic audio, transcribe those dialogs with internal speech acknowledgment models, and utilize natural language processing algorithms to understand every conversation. They use this universe of individually discussion to identify what each representative– and the company at large– is succeeding and what they aren’t, all with the objective of making every call a success. Dialpad had worked with a rival of Appen for six months however were having trouble reaching a precision threshold to make their designs a success. It took simply a couple weeks for the change to flourish for Dialpad and to produce the transcription and NLP training data they required to make their models a success. (Click here to read the complete case study).
Image Annotation.
Image annotation is crucial for a large range of applications, consisting of computer system vision, robotic vision, facial recognition, and options that rely on device learning to translate images. To train these services, metadata should be designated to the images in the form of identifiers, captions, or keywords. From computer system vision systems used by self-driving cars and machines that select and arrange fruit and vegetables, to healthcare applications that auto-identify medical conditions, there are many use cases that need high volumes of annotated images. Image annotation increases precision and accuracy by successfully training these systems data annotator.
Appen image annotation facial recognition.
Real Life Use Case: Adobe Stock Leverages Massive Asset Profile to Make Customers Happy.
Among Adobe’s flagship offerings is Adobe Stock, a curated collection of top quality stock images. The library itself is terribly big: there are over 200 million properties (consisting of more than 15 million videos, 35 million vectors, 12 million editorial assets, and 140 million photos, illustrations, design templates, and 3D assets). Every one of those assets needs to be visible. Appen supplied highly precise training data to develop a design that could appear these subtle qualities in both their library of over a hundred million images, along with the numerous countless new images that are published every day. That training data powers designs that assist Adobe serve their most important images to their massive customer base. Instead of scrolling through pages of comparable images, users can find the most helpful ones quickly, releasing them up to start producing powerful marketing materials. (Read the complete case study here).
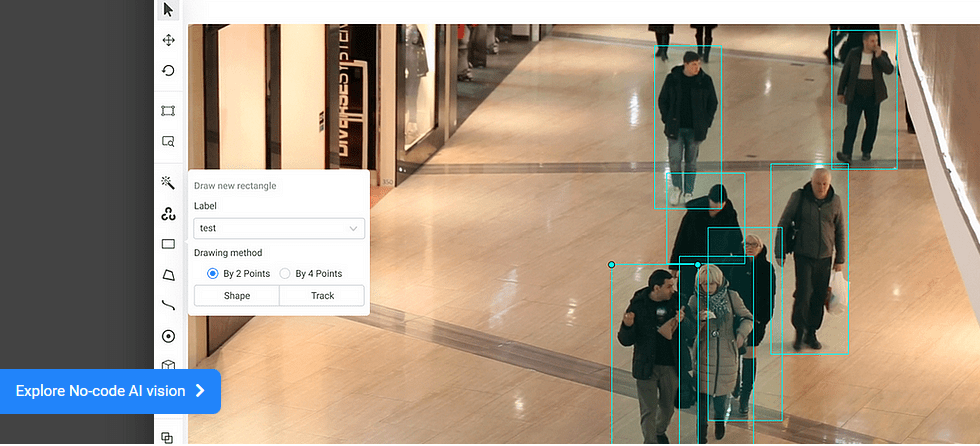
Video Annotation.
Human-annotated data is the key to effective artificial intelligence. Humans are merely better than computer systems at managing subjectivity, understanding intent, and coping with ambiguity. For instance, when determining whether an online search engine outcome is relevant, input from many people is needed for consensus. When training a computer vision or pattern recognition option, human beings are required to recognize and annotate specific data, such as detailing all the pixels including trees or traffic signs in an image. Utilizing this structured information, makers can find out to recognize these relationships in testing and production.
Real World Use Case: HERE Technologies Creates Data to Fine-Tune Maps Faster Than Ever.
With an objective of creating three-dimensional maps that are accurate to a few centimeters, HERE has remained an innovator in the space because the mid- ’80s, giving numerous businesses and organizations detailed, precise and actionable location information and insights. HERE has an enthusiastic objective of annotating tens of countless kilometers of driven roadways for the ground fact data that powers their sign-detection models. Parsing videos into images for that goal, however, is just untenable. Our Machine Learning assisted Video Object Tracking option provided an ideal service to this lofty aspiration. That’s since it integrates human intelligence with machine finding out to considerably increase the speed of video annotation. (Click here to read the complete case study).
What Appen Can Do For You.
At Appen, our data annotation experience spans over twenty years. By integrating our human-assisted technique with machine-learning assistance, we give you the top quality training information you need. Our text annotation, image annotation, audio annotation, and video annotation will give you the self-confidence to release your AI and ML models at scale. Whatever your information annotation needs might be, our platform and managed service team are waiting to help you in both releasing and preserving your AI and ML projects.
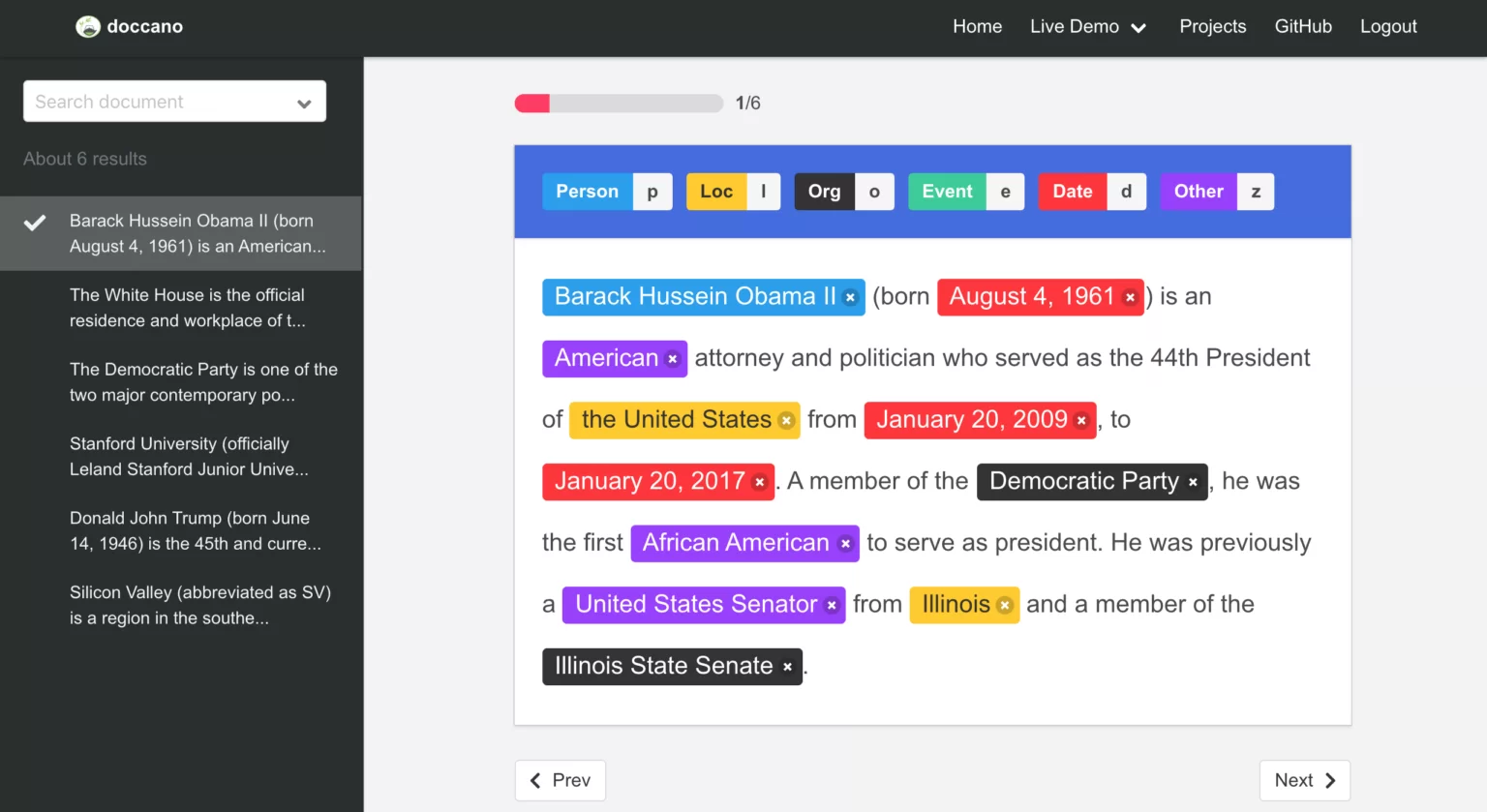
Annotated information is an important part of different artificial intelligence and expert system (AI) applications. It is likewise one of the most time-consuming and labor-intensive parts of AI/ML tasks. Information annotation is among the leading constraints of AI execution for companies.
Tech leaders and designers require to focus on improving information annotation for their data-hungry digital options. To correct that, we suggest getting a thorough understanding of data annotation.
Our research study covers the following:.
What is data annotation?
Why it matters?
What its techniques/types are?
What are some crucial difficulties of annotating information?
What are some best practices for information annotation?
What is information annotation?
Data annotation is the process of labeling data with pertinent tags to make it simpler for computer systems to understand and interpret. This information can be in the form of images, text, audio, or video, and information annotators need to identify it as properly as possible. Information annotation can be done by hand by a human or automatically utilizing sophisticated maker finding out algorithms and tools. To read more about automated information annotation/labeling, have a look at this fast read.
For monitored artificial intelligence, labeled datasets are crucial because ML designs require to understand input patterns to process them and produce accurate outcomes. Supervised ML designs (see figure 1) train and learn from properly annotated data and solve problems such as:.
Category: Assigning test data into particular classifications. For example, predicting whether a client has an illness and appointing their health information to “illness” or “no illness” categories is a classification issue.
Regression: Establishing a relationship between reliant and independent variables. Approximating the relationship between the budget for advertising and the sales of a product is an example of a regression issue.
Figure 1: Supervised Learning Example.
The image shows the supervised learning example. The training dataset has all type of fruits with different labels. the test set just has 2 types of fruit.
Source: Diego Calvo.
For example, training maker discovering designs of self-driving cars and trucks include annotated video information. Specific things in videos are annotated, which enables devices to predict the motions of things.
Other terms to explain information annotation consist of information labeling, information tagging, data category, or machine learning training information generation.
Why does information annotation matter?
Annotated information is the lifeline of supervised knowing models given that the performance and accuracy of such models depend on the quality and amount of annotated information. Devices can not see images and videos as we do. Data annotation makes the different information types machine-readable. Annotated data matters since:.
Artificial intelligence models have a wide array of crucial applications (e.g., health care) where incorrect AI/ML models can be dangerous.
Finding high-quality annotated data is one of the primary obstacles of structure precise machine-learning designs.
Please see our data labeling post for more on why data annotation/data labeling matters and how to pick the ideal data annotation partner.
Sponsored.
Data collection is a prerequisite of data annotation, and it must be done right to guarantee the general quality of the dataset. Clickworker offers both data collection and annotation services through a crowdsourcing platform. Their international labor force of over 4 million registered information collectors offers diverse and scalable datasets and image annotation services.
For more, have a look at our:.
Short article on information collection.
Data-driven list of information collection/harvesting services.
What are the different kinds of information annotation?
Different information annotation techniques can be used depending on the machine learning application. A few of the most common types are:.
1. Text annotation.
Text annotation trains devices to much better comprehend the text. For example, chatbots can determine users’ requests with the keywords taught to the device and offer options. If annotations are inaccurate, the device is not likely to offer a beneficial service. Better text annotations provide a much better client experience. During the data annotation process, with text annotation, some particular keywords, sentences, and so on, are appointed to data points. Comprehensive text annotations are important for precise machine training. Some types of text annotation are:.
1.1. Semantic annotation.
Semantic annotation (see figure 2) is the procedure of tagging text documents. By tagging documents with relevant principles, semantic annotation makes unstructured material simpler to find. Computers can interpret and check out the relationship in between a specific part of metadata and a resource explained by semantic annotation.
Figure 2: Semantic Annotation Example.
The image reveals an example of tagged words in a text document.
Source: Articles Hubspot.
1.2. Objective annotation.
For example, the sentence “I want to talk with David” shows a request. Intent annotation analyzes the needs behind such texts and categorizes them, such as requests and approvals.
1.3. image annotation.
Sentiment annotation (see Figure 3) tags the feelings within the text and assists makers recognize human emotions through words. Machine learning designs are trained with belief annotation data to discover the true feelings within the text. For instance, by reading the comments left by consumers about the items, ML models understand the mindset and emotion behind the text and then make the relevant labeling such as positive, unfavorable, or neutral.
Figure 3: Sentiment Annotation Example.
The image reveals the process of identifying texts in documents.
Source: Sentiment Annotation– Quick Start Guide.
2. Text classification.
Text categorization designates categories to the sentences in the document or the whole paragraph in accordance with the topic. Users can quickly discover the info they are searching for on the site.
3. Image annotation.
Image annotation is the procedure of identifying images (see figure 4) to train an AI or ML model. For example, a machine learning model gets a high level of understanding like a human with tagged digital images and can analyze the images it sees. With data annotation, things in any image are labeled. Depending on the usage case, the variety of labels on the image may increase. There are four essential types of image annotation:.
3.1. Image classification.
Initially, the device trained with annotated images then determines what an image represents with the predefined annotated images.
3.2. Object recognition/detection.
Object recognition/detection is a further variation of image category. It is the correct description of the numbers and exact positions of entities in the image. While a label is appointed to the whole image in image category, object acknowledgment labels entities independently. For example, with image classification, the image is identified as day or night. Object acknowledgment individually tags various entities in an image, such as a bicycle, tree, or table.
3.3. Segmentation.
Division is a more advanced kind of image annotation. In order to analyze the image more quickly, it divides the image into several sections, and these parts are called image things. There are three kinds of image division:.
Semantic segmentation: Label similar objects in the image according to their homes, such as their size and location.
Circumstances division: Each entity in the image can be labeled. It defines the homes of entities such as position and number.
Panoptic segmentation: Both semantic and instance segmentations are utilized by combining.
Figure 4: Image annotation example.
This image demonstrates how image annotation varies from other information annotation types.
Source: Medium.
4. Video annotation.
Video annotation is the process of mentor computer systems to acknowledge objects from videos. Image and video annotation are kinds of information annotation approaches that are carried out to train computer system vision (CV) systems, which is a subfield of artificial intelligence (AI).
Video annotation for a store security system:.
Click on this link for more information about video annotation.
5. Audio annotation.
Audio annotation is a kind of information annotation that includes categorizing elements in audio information. Like all other kinds of annotation (such as image and text annotation), audio annotation requires manual labeling and specialized software. Solutions based on natural language processing (NLP) depend on audio annotation, and as their market grows (predicted to grow 14 times in between 2017 and 2025), the demand and importance of quality audio annotation will grow as well.
Audio annotation can be done through software that permits information annotators to label audio information with pertinent words or phrases. For instance, they might be asked to identify a noise of a person coughing as “cough.”.
Audio annotation can be:.
In-house, completed by that company’s employees.
Outsourced (i.e., done by a third-party business.).
Crowdsourced. Crowdsourced data annotation includes using a large network of information annotators to identify data through an online platform.
Learn more about audio annotation.
6. Industry-specific data annotation.
Each industry uses data annotation in a different way. Some industries use one type of annotation, and others utilize a combination to annotate their data. This section highlights a few of the industry-specific types of information annotation.
Medical data annotation: Medical information annotation is used to annotate information such as medical images (MRI scans), EMRs, and clinical notes, and so on. This type of information annotation assists develop computer system vision-enabled systems for disease medical diagnosis and automatic medical information analysis.
Retail information annotation: Retail data annotation is used to annotate retail information such as product images, customer information, and belief data. This type of annotation helps create and train accurate AI/ML designs to determine the belief of clients, product suggestions, and so on.
Finance data annotation: Finance information annotation is used to annotate data such as financial files, transactional information, etc. This kind of annotation assists develop AI/ML systems, such as fraud and compliance problems detection systems.
Automotive data annotation: This industry-specific annotation is used to annotate data from self-governing vehicles, such as information from cams and lidar sensing units. This annotation type helps establish designs that can identify objects in the environment and other data points for self-governing lorry systems.
Industrial information annotation: Industrial data annotation is utilized to annotate information from industrial applications, such as manufacturing images, maintenance information, safety information, quality assurance, etc. This kind of data annotation assists develop models that can identify abnormalities in production procedures and make sure worker security.
What is the distinction in between information annotation and information labeling?
Information annotation and information labeling mean the same thing. You will come across short articles that try to discuss them in different ways and comprise a difference. For example, some sources claim that information labeling is a subset of data annotation where data aspects are assigned labels according to predefined guidelines or criteria. Nevertheless, based on our conversations with vendors in this area and with data annotation users, we do not see significant differences in between these concepts.
What are the primary obstacles of data annotation?
Expense of annotating data: Data annotation can be done either manually or immediately. However, by hand annotating information needs a lot of effort, and you likewise require to maintain the quality of the information.
Accuracy of annotation: Human errors can cause poor data quality, and these have a direct impact on the prediction of AI/ML models. Gartner’s study highlights that bad data quality costs companies 15% of their profits.
What are the very best practices for information annotation?
Start with the proper data structure: Focus on developing data labels that are specific enough to be useful but still basic enough to catch all possible variations in information sets.
Prepare comprehensive and easy-to-read directions: Develop data annotation guidelines and best practices to ensure information consistency and accuracy throughout various information annotators.
Optimize the amount of annotation work: Annotation is costlier and less expensive alternatives need to be taken a look at. You can work with an information collection service that uses pre-labeled datasets.
Collect information if required: If you don’t annotate enough information for machine learning models, their quality can suffer. You can deal with data collection companies to gather more data.
Take advantage of outsourcing or crowdsourcing if information annotation requirements end up being too large and time-consuming for internal resources.
Assistance human beings with devices: Use a mix of artificial intelligence algorithms (data annotation software application) with a human-in-the-loop technique to help people focus on the hardest cases and increase the variety of the training data set. Identifying information that the device finding out design can properly process has actually limited worth.
Concentrate on quality:.
Routinely test your information annotations for quality control functions.
Have several information annotators review each other’s work for precision and consistency in identifying datasets.
Stay certified: Carefully consider privacy and ethical problems when annotating sensitive information sets, such as images including individuals or health records. Absence of compliance with regional rules can damage your business’s credibility.
By following these data annotation best practices, you can ensure that your information sets are precisely labeled and accessible to information scientists and sustain your data-hungry jobs.
You can likewise inspect our information annotation services and video annotation tools lists to select the fit that best fits your annotation needs.
For more extensive understanding of data collection, do not hesitate to download our detailed whitepaper:.
What is Data Annotation?
A brief definition of Data Annotation.
Share on Facebook.
Information annotation is simply the procedure of labeling details so that devices can use it. It is especially beneficial for supervised machine learning (ML), where the system relies on labeled datasets to process, comprehend, and learn from input patterns to arrive at wanted outputs.
In ML, information annotation occurs prior to the information gets fed to a system. The procedure can be likened to utilizing flashcards to teach children. A flashcard with the picture of an apple and the word “apple” would inform the children how an apple looks and how the word is spelled. In that example, the word “apple” is the label.
Other fascinating terms …
What is Machine Learning (ML)?
What is Supervised Learning?
Read More about “Data Annotation”.
Information annotation is an important part of supervised ML. Without it, makers can’t properly analyze inputs to provide the desired outputs. In this section we will cover the different kinds of information annotation, and a number of essential use cases. You can also examine Data Annotation Guide: Everything a Beginner Needs to Know to find out more about data annotation.
Types of Data Annotation in ML.
Data can be annotated in different ways for a maker’s use, consisting of:.
1. Semantic Annotation.
This technique includes labeling different principles with text like “things,” “individuals,” and “names.” Semantic annotation is utilized to train chatbots and enhance the importance of online search engine results. Enjoy this video for additional information.
2. Image and Video Annotation.
Identifying images and videos enable machines to understand images and video content. Frequently, developers use bounding boxes to inform computers what to focus on so they can determine specific objects. Image and video annotation is commonly applied to autonomous automobiles and e-commerce item listing.
3. Text Classification or Categorization.
This method describes the process of drawing out generic tags from unstructured text. The generic tags come from a set of predefined categories. Text category or classification assists users quickly look for information and browse within a website or an application.
Data Annotation Use Cases.
Data annotation is useful in:.
1. Improving the Quality of Search Engine Results for Multiple User Types.
Online search engine require to offer users with comprehensive information. Their algorithms must process high volumes of labeled datasets to give the right answer to do that. Take, for example, Microsoft’s Bing. Since it caters to multiple markets, the vendor needs to make sure that the results the search engine would provide would match the user’s culture, line of business, and so on.
2. Refining Local Search Evaluation.
While search engines cater to a global audience, vendors also have to make sure that they give users localized results. Data annotators can help with that by labeling information, images, and other content according to geolocation.
3. Enhancing Social Media Content Relevance.
Like search engines, social media platforms also need to provide customized content recommendations to users. Data annotation can help developers classify and categorize content for relevance. An example would be categorizing which content a user is likely to consume or appreciate based on his/her viewing habits and which he/she would find relevant based on where he/she lives or works.
Data annotation is time-consuming and tedious. Thankfully, artificial intelligence (AI) systems are now available to automate the process.
WHAT IS DATA ANNOTATION